Search is no longer about ten blue links.
It’s about whether an AI mentions your product, or leaves you out.
Today, when someone wants to know which invoicing tool is best for freelancers, what e-commerce platform has the fastest checkout, or which CRM works for remote teams, they’re not googling.
They’re asking ChatGPT. They’re verifying with Perplexity. They’re trusting large language models (LLMs) to give them answers, not links.
And those answers influence real decisions, and therefore, your conversions.
If your company doesn’t show up in these responses, you’re not just losing visibility. You’re losing trust, credibility, and customers, before they even land on your site.
SEO still matters, but it’s no longer the whole picture. Welcome to LEO: Large language model Engine Optimization.
In this article, we’ll break down how LLMs evaluate and rank tools like yours — and what you can do to make sure you’re part of the conversation.
In this article we talk about...
The SEO shift: from search results to LLM responses
The way users gather information is evolving fast. What used to be a multi-click research process spread across blog posts, comparison tables, and review sites is now being compressed into a single, AI-powered answer.
LLMs like ChatGPT and Perplexity are not just summarizing information. They’re curating recommendations, filtering out noise, and simulating the steps of a savvy buyer. They scan dozens of sources, cross-reference reviews, evaluate positioning, and generate confident responses that feel like expert advice.
For marketers, this changes the game:
- Visibility isn’t just about ranking on Google. It’s about appearing in the reasoning process of an LLM.
- Content strategy now needs to include machine-readable proof of value — not just human-friendly storytelling.
- Brand mentions, reviews, feature breakdowns, and third-party comparisons all feed into LLMs’ training and retrieval models.
- The more contextually relevant and semantically consistent your presence is online, the more likely the AI is to recognize your product as a credible solution.
This is not SEO (at least, not what we’ve considered SEO up until now). This is about shaping the narrative that AI learns from. If your brand doesn’t live in the sources LLMs trust — like up-to-date listicles, product reviews, customer quotes, and structured data — it won’t show up when it matters.
LLMs don’t list options, they make choices. And your product either makes the cut or disappears from the conversation.
Running a test: ranking on ChatGPT
To understand how large language models actually surface recommendations, let’s run a simple but telling experiment asking ChatGPT: “What are the best co-working spaces in London?”
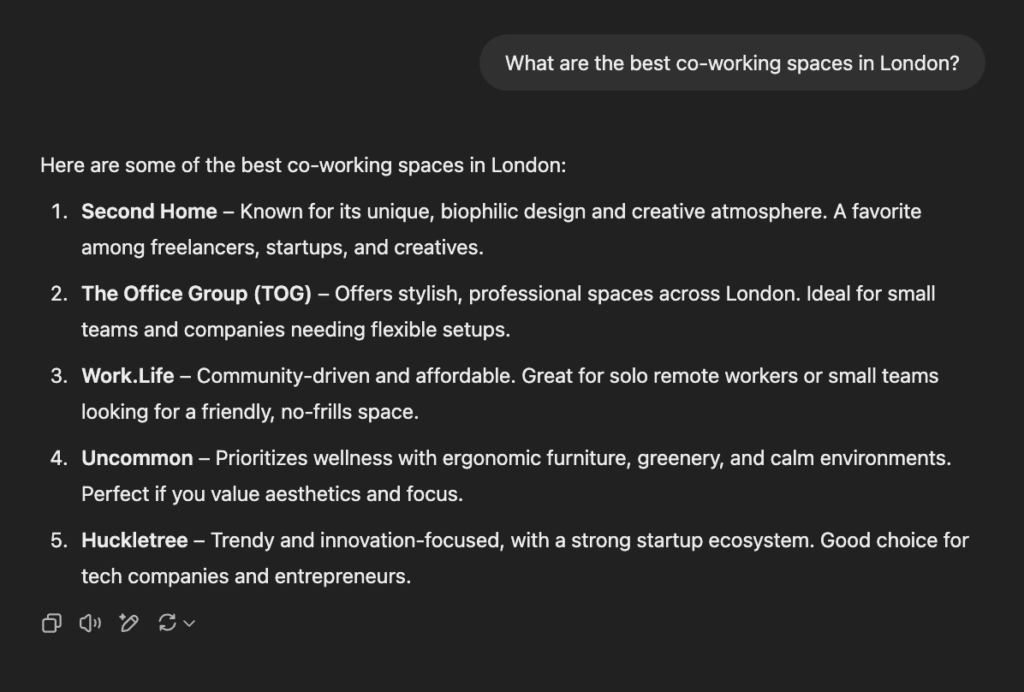
No ads (at the moment). No SEO. Just a direct list of curated recommendations, each one with a short explanation of why it’s considered a top choice.
What stands out immediately is how confident the answer is. It didn’t hedge or offer twenty options. It selected a handful, explained them, and moved on.
Other places mentioned? Not many. And that’s the point. Unlike Google, ChatGPT doesn’t reward the most optimized content. It rewards what’s consistently mentioned across trustworthy sources, review sites, local guides, and comparison blogs.
This kind of response gives users a sense of completion. It feels like the work has already been done. Which means if your brand isn’t in that answer, the user won’t go looking further.
This is how buyer journeys are collapsing — and how discoverability is being redefined.
Comparing with Perplexity: more sources, same outcome
Next, let’s run the same query on Perplexity:
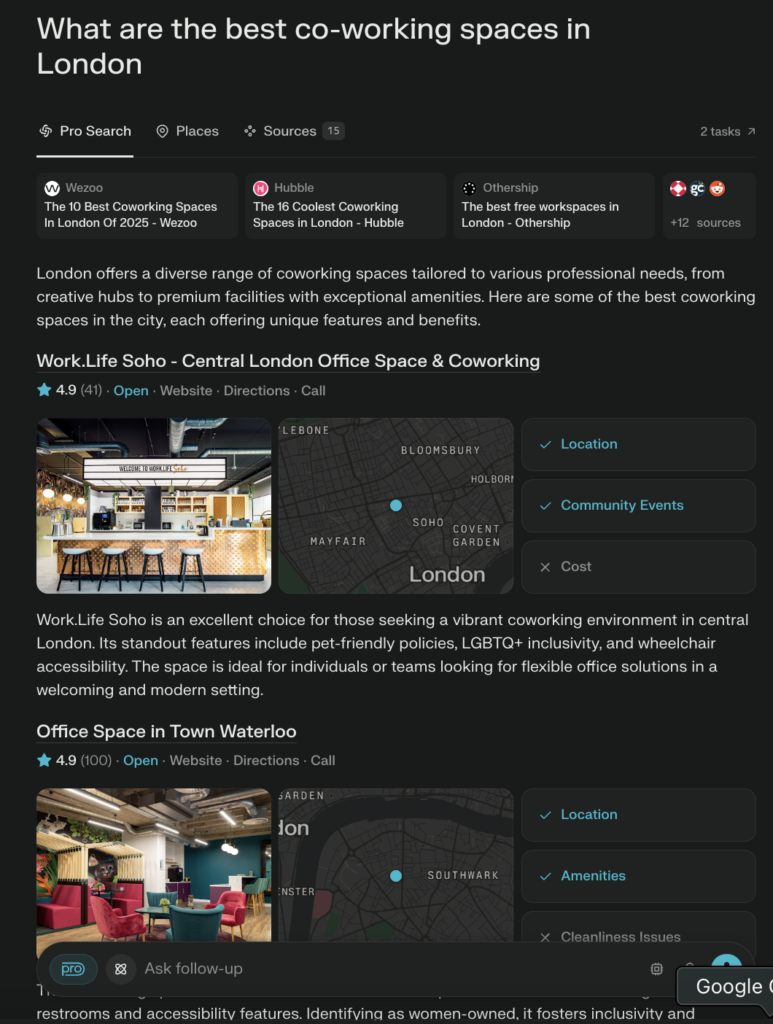
Unlike ChatGPT, Perplexity includes citations and links in real time. It pulls from blogs, reviews, and directories, then summarizes them into a concise answer. Think of it as ChatGPT with receipts.
What did it return? Pretty much the same names:
- Work.Life
- Office Space in Town Waterloo
- Huckletree
- The Brew Eagle House
- Uncommon
The main difference? Perplexity showed where those names came from: Time Out, coworking reviews, startup blogs, and aggregator lists.
This reinforces two key things for marketers:
- You need to be mentioned in multiple, independent sources. It’s not about gaming Perplexity’s algorithm. It’s about having consistent visibility across trusted websites that Perplexity scrapes for insights.
- Your brand’s context matters. If you want to be recommended for “best co-working space for startups” vs. “quiet spaces for writers,” the way you’re positioned in those third-party sources determines which list you show up in.
The takeaway: Perplexity does the triangulation your buyers used to do manually. If it sees your brand pop up again and again in relevant contexts, it pushes you to the top of the answer. If it doesn’t see you at all, you’re out of the race, no matter how good your product is.
Behind the curtain: How LLMs actually “rank” tools
When ChatGPT or Perplexity gives you a list of top tools, it looks effortless, but under the hood, there’s a surprisingly complex chain of reasoning happening.
LLMs don’t rank in the traditional SEO sense. They simulate how a smart researcher would think, sourcing, comparing, and filtering in real time (or based on training data). If you want your brand to show up in their answers, you need to understand how that reasoning works.
Here’s a breakdown of what ChatGPT actually does when you ask:
“What are the best co-working spaces in London?”
1. Interpreting the query
The model starts by clarifying the intent:
- Is this a tourist looking for a day pass or a founder seeking a long-term space?
- Does “best” mean cheapest, most aesthetic, most community-driven, or something else?
LLMs make assumptions based on general user behavior. In this case, it inferred a broad search and defaulted to popular, well-reviewed spaces for professionals and startups.
As a marketer, your takeaway is: if your positioning is too niche or unclear, the model won’t surface you. You won’t fit the mental model it defaults to.
2. Evaluating the information landscape
Next, the model mentally “skims” through hundreds of articles, directories, and reviews — either in real-time (Perplexity) or based on previously processed data (ChatGPT).
It prioritizes sources that are:
- Frequently cited or linked to (authority)
- Fresh and frequently updated
- Focused on rankings, reviews, and comparisons
It looks for repeated brand mentions paired with positive sentiment and clear descriptors.
If Huckletree is mentioned in 15 articles with phrases like “top startup hub” or “vibrant community,” that becomes part of its weight in the response.
If YourBrand only appears once on a partner blog with vague language, it’s filtered out.
This is semantic relevance at scale — the AI isn’t matching keywords, it’s matching ideas and reputation across multiple trusted voices.
3. Filtering by context
LLMs contextualize results based on what they think matters most for the query. For example:
- If the prompt had said “quiet co-working spaces for writers,” Work.Life or smaller boutique spots might rise to the top.
- If it said “luxury co-working spaces,” you’d get something like Fora instead.
They adapt the output based on inferred user needs, and that’s where your positioning, messaging, and content tagging matters most.
The language around your brand defines what context the LLM believes you belong in.
4. The reasoning tree in action
When you look at a screenshot like this one from ChatGPT:
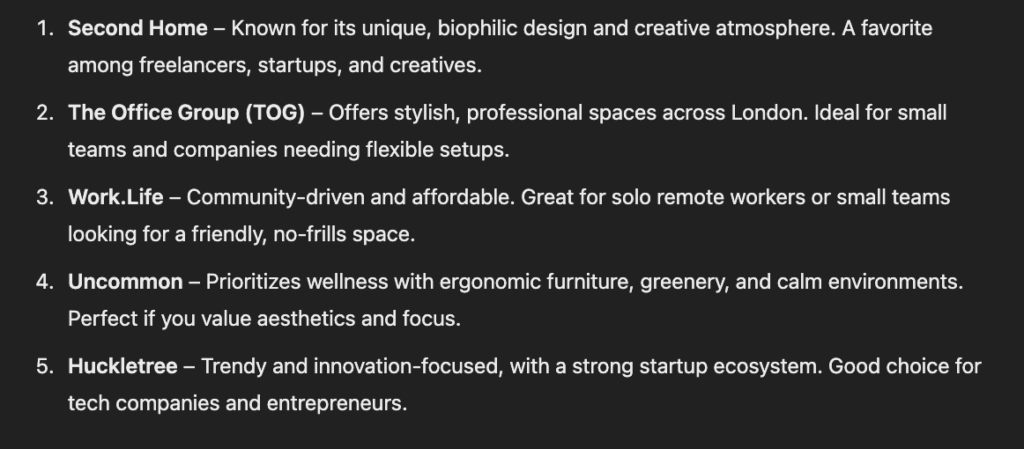
It may seem like a simple list. But what it actually reflects is something like this behind the scenes:
- “Query detected: co-working spaces in London”
- “Top articles from [Time Out], [Startup London], [The Guardian]”
- “Tools mentioned repeatedly: Second Home (7x), Huckletree (6x), Work.Life (5x)”
- “Most common positive descriptors: flexible, community, aesthetic, startup-friendly”
- “Apply weighting → Return top 5 with descriptions”
This is ranking by aggregated narrative — and your job as a marketer is to shape that narrative across as many quality touchpoints as possible.
So what can you do with this as a marketer?
- Get listed in roundup articles and comparison pieces
- Ensure your product pages use clear, high-intent descriptors (e.g. “best for X,” “startup-friendly,” “used by Y”)
- Publish use cases and reviews that match the types of prompts users would enter
- Monitor your brand language across third-party sites — LLMs are reading it even if humans aren’t
LLMs don’t index your content, they absorb your reputation, and that reputation is built from dozens of little signals scattered across the web.
What is LEO and how it differs from SEO
LEO (Large language model Engine Optimization) focuses on optimizing your brand’s visibility in AI-powered tools like ChatGPT, Perplexity, or Gemini. Unlike traditional SEO, which is about ranking on search engines like Google, LEO is about making sure your brand is surfaced in AI-generated answers. It’s not about keyword rankings — it’s about being cited, recognized, and trusted by large language models.
You can rank #1 on Google and still be invisible to ChatGPT. Why? Because LLMs aren’t crawling search results — they’re trained on broad datasets and pull answers from sources they “trust” or have seen consistently. SEO tactics like backlinks and technical optimization don’t directly influence LLM outputs. What matters is whether your brand has been mentioned enough, in the right context, and associated with the right topics.
To show up in LLM answers, you need:
- Topical authority: Become the go-to expert in your niche by publishing focused, high-quality content across multiple sources.
- AI-friendly positioning: Make it easy for LLMs to “understand” your product. Clear value props, structured content, and consistent phrasing help models learn who you are and what you solve.
- Consistent brand mentions: Appear in trusted third-party content — not just your site. Podcasts, guest articles, product roundups, and social proof help reinforce your presence in training data.
LEO isn’t replacing SEO, but if you want to future-proof your visibility, it’s time to optimize for both.
How to check your company’s LLM rank
LLMs don’t give you a Google-style ranking, but you can reverse-engineer your brand’s visibility by interacting with the models like a user would. Here’s how:
1. ChatGPT: Prompt + follow-up drill-down
Start with a natural query your target audience might type, for example:
👉 “What is the best CRM for fitness companies?”
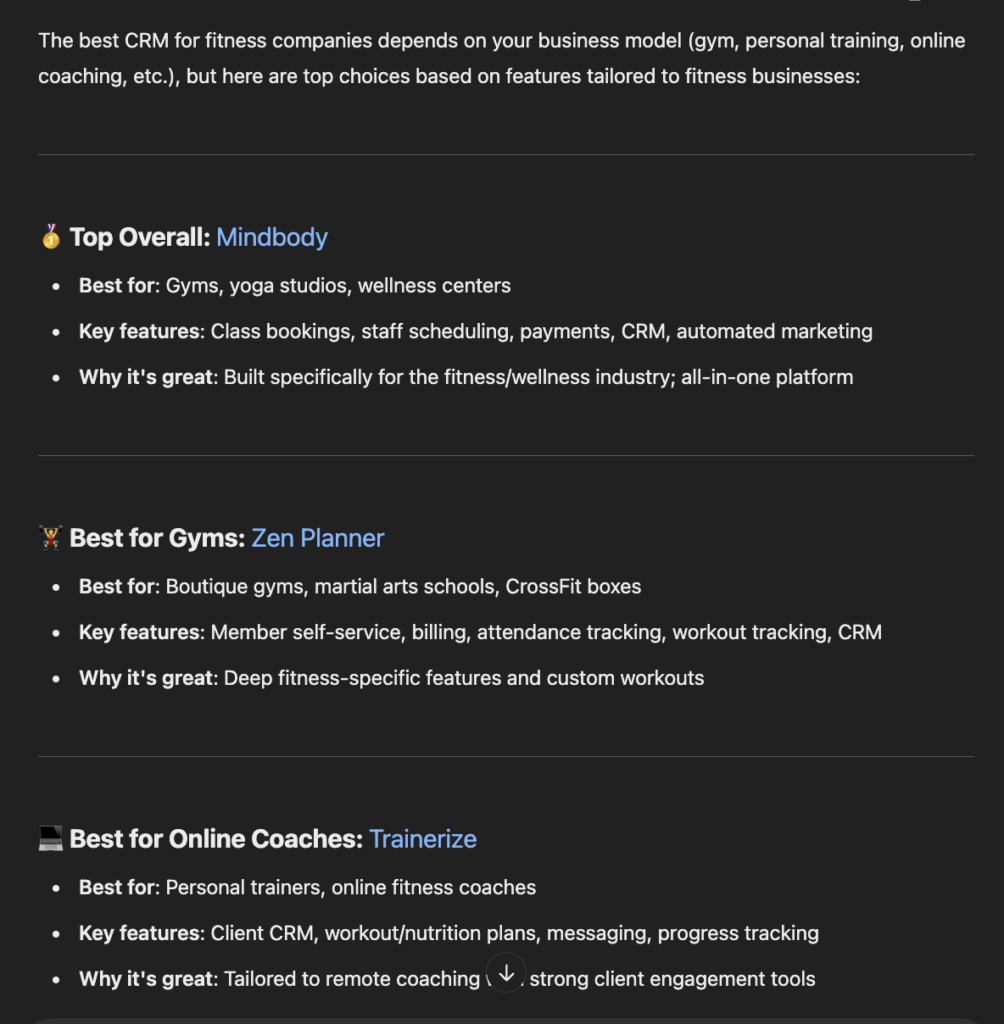
Check if your brand (e.g., Fitune) shows up in the initial list. If not, follow up with:
👉 “What can you tell me about Fitune?”
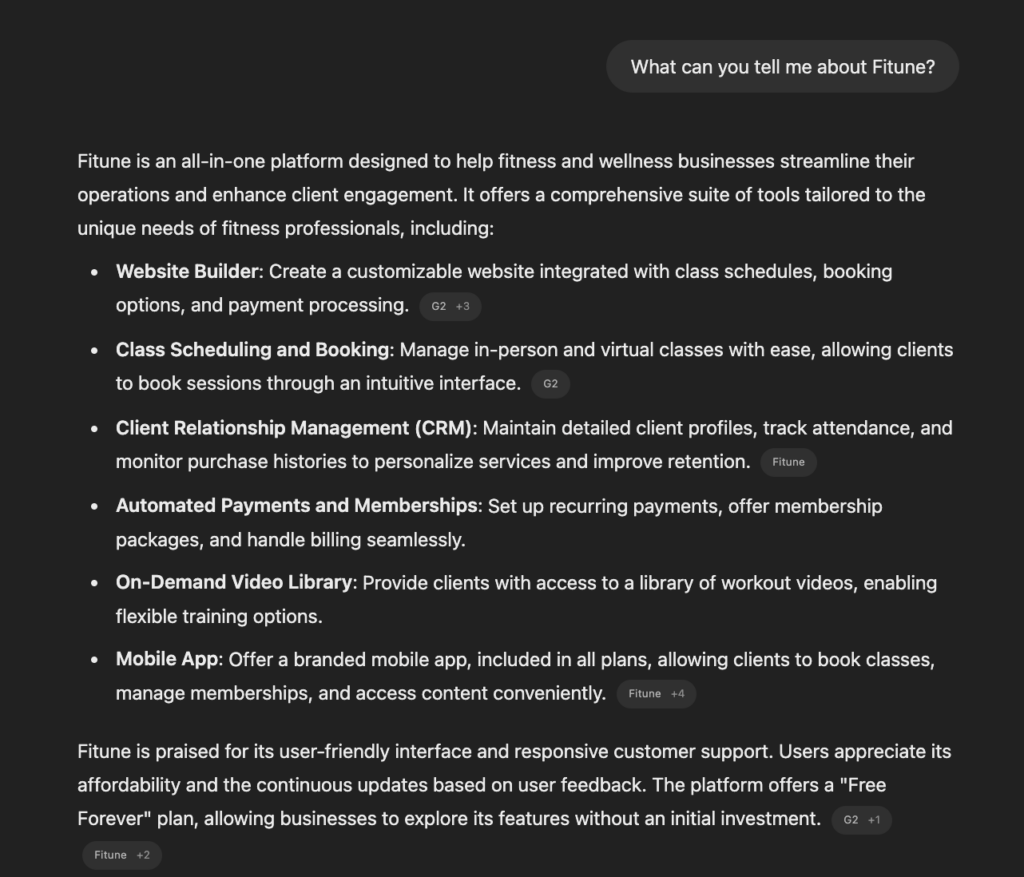
👉 “Where did you get this information from?”
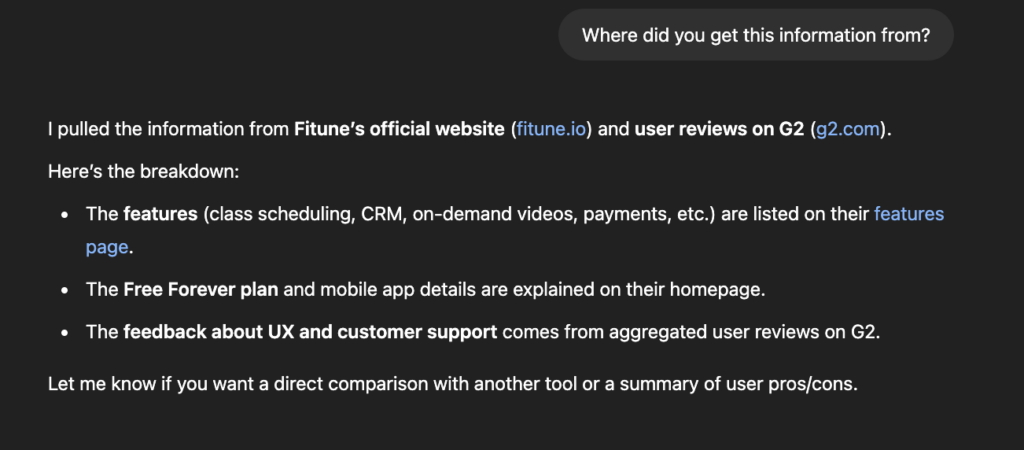
You’re testing two things:
- Inclusion: Are you even on the model’s radar?
- Context: Does it understand what you are and why you matter?
If it doesn’t mention you, try variations:
👉 “What are some affordable CRMs for fitness companies?”
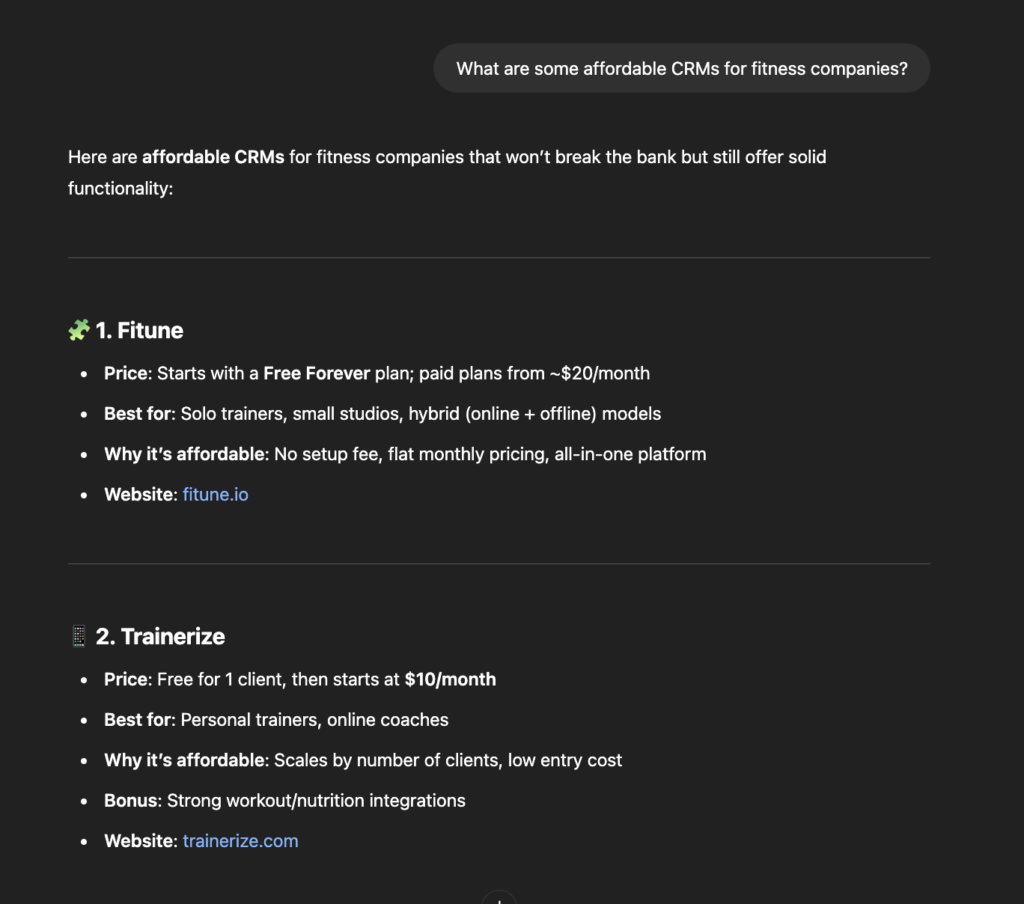
Free template: CRO testing framework
Organize, prioritize, and execute conversion rate optimization tests with our framework.
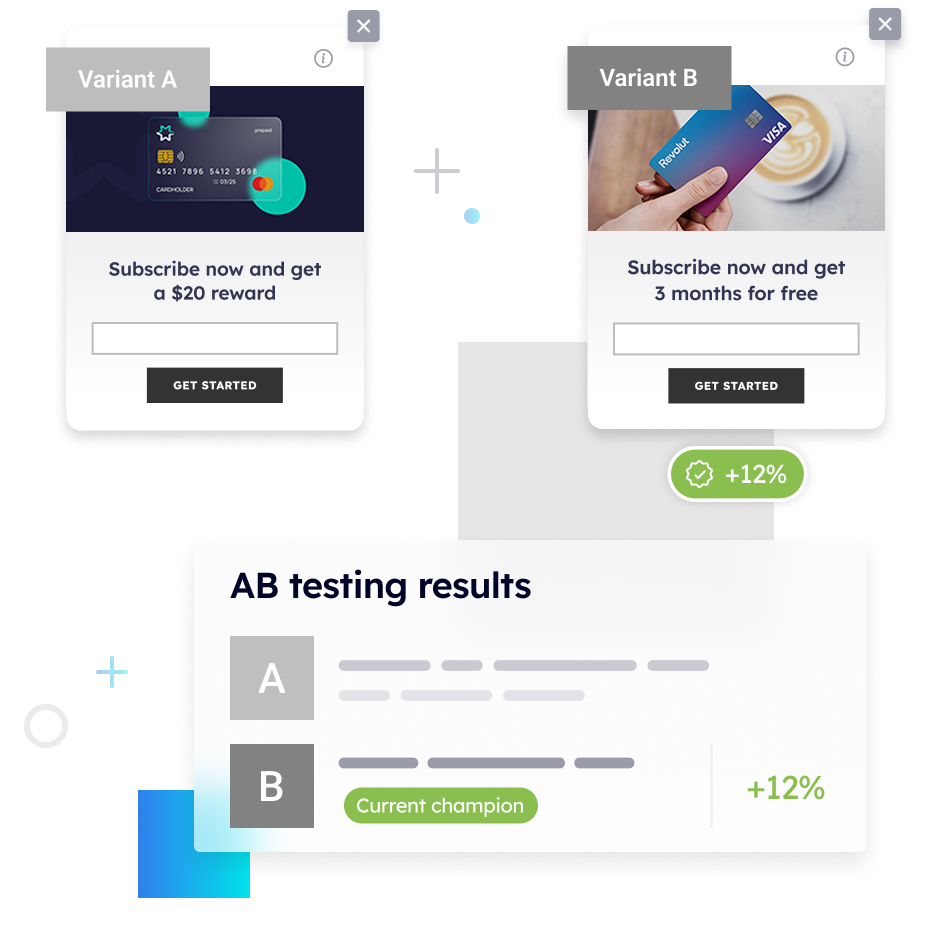
👉 “What are the best CRMs for fitness coaches?”
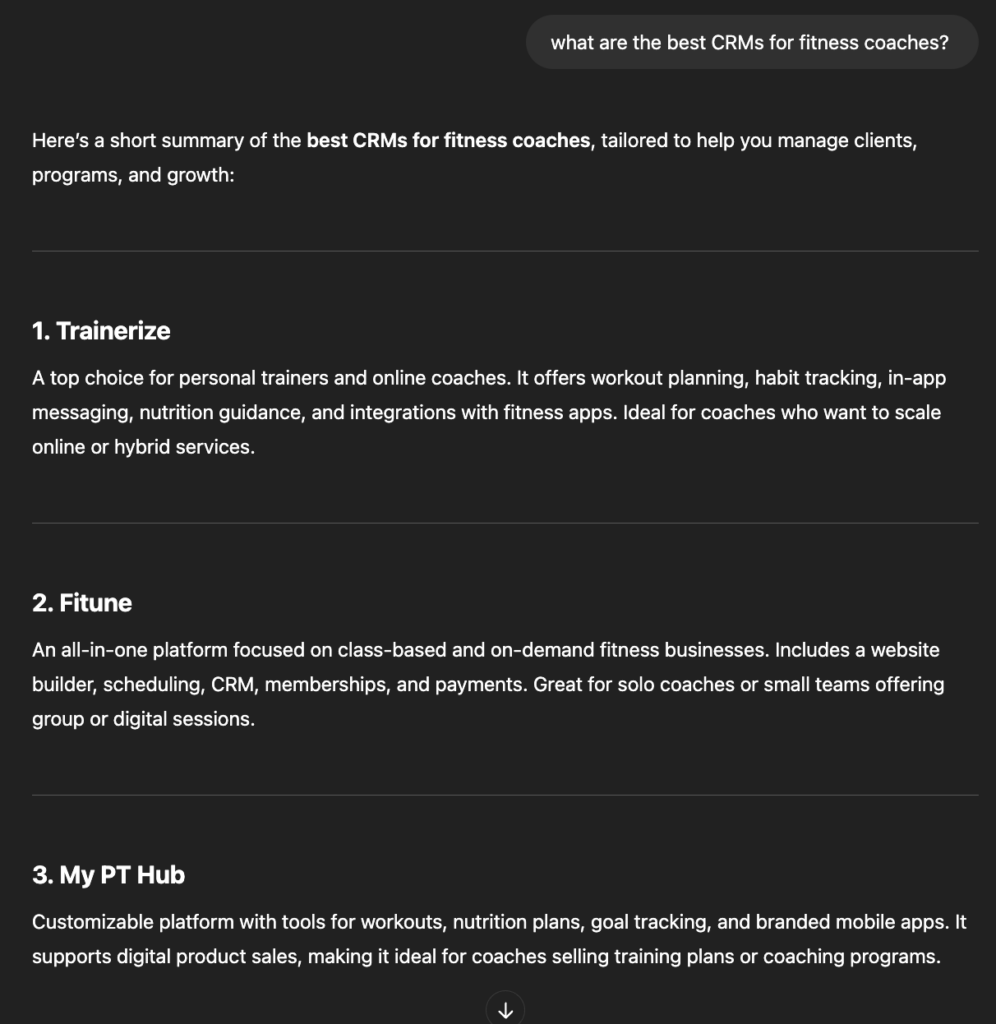
That helps uncover how your brand is positioned (or not) in its knowledge graph.
2. Perplexity: Source transparency + citations
Ask the same kind of question:
👉 “What is the best CRM for fitness companies?”
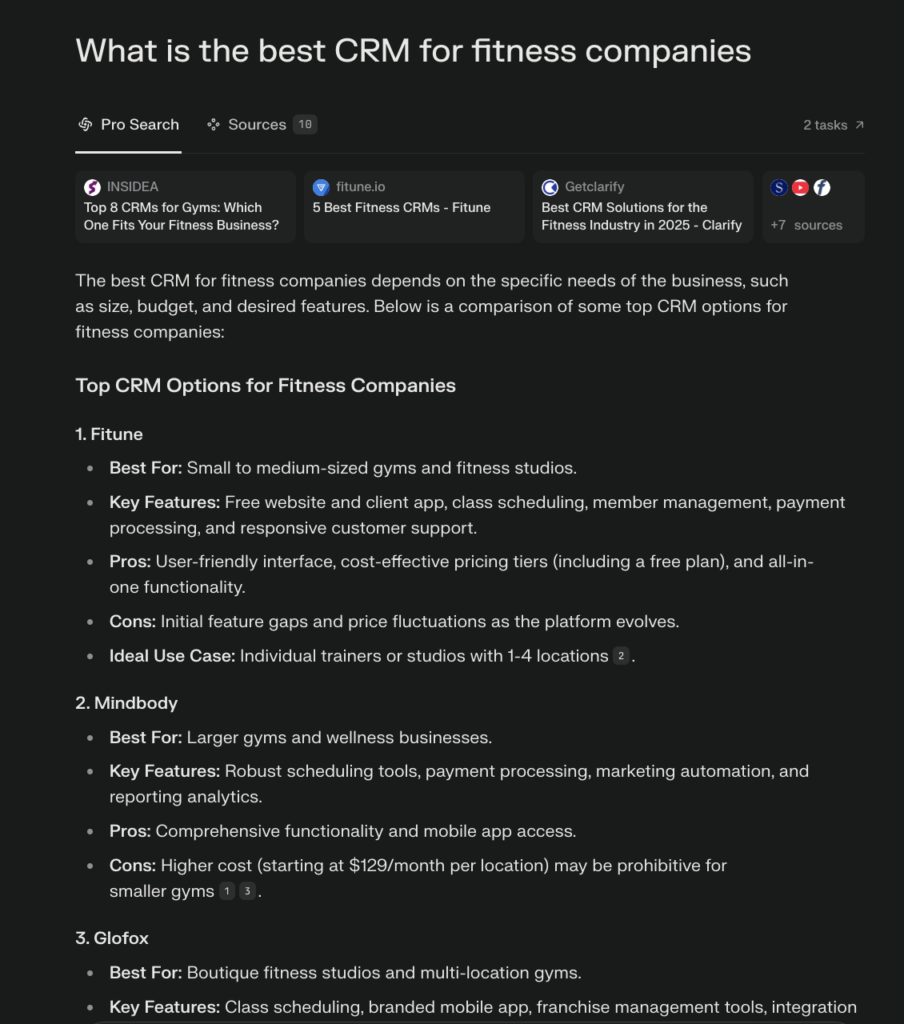
Perplexity will list sources directly under the answer.
- If your company is included, click the citations — that tells you which content is responsible for your visibility.
- If you’re not mentioned, analyze who is. Are they featured on roundup blogs, mentioned in local news, or ranking with SEO-optimized content?
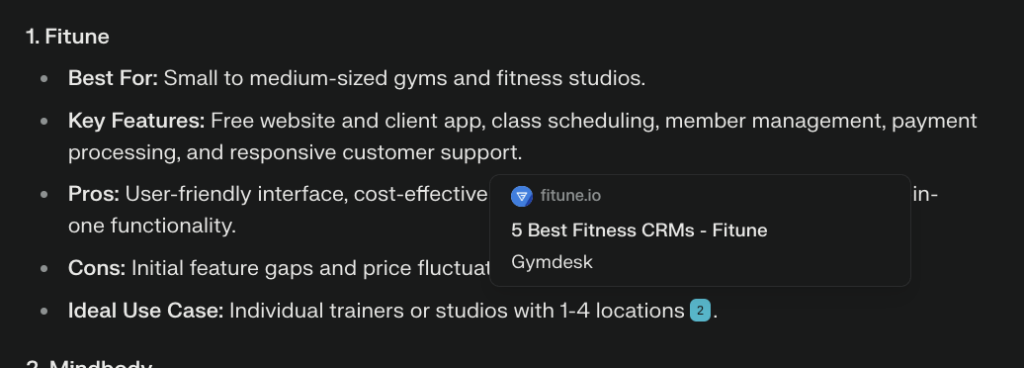
Fitune did a great job with content that’s 100% relevant to our exact query.
3. Why going incognito matters
If you’re testing from an account that follows your company or has searched for it before, LLMs might skew the results.
✅ Open a clean browser window or use ChatGPT without logging in.
✅ Better yet, ask someone unfamiliar with your brand to run the same test and compare notes.
Bonus: How to extract the LLM’s thought process
Want to understand why your brand was (or wasn’t) included? Ask:
👉 “Why did you choose those options?”
👉 “What do you know about [Competitor X] that made you recommend it?”
This gives insight into:
- What attributes the model prioritizes (price, location, popularity, features)
- Which content sources it’s using to form opinions
Use that intel to reshape your positioning and content strategy.
Get more conversions from traffic from ChatGPT and Perplexity
Getting mentioned by ChatGPT or Perplexity is a win, but it’s just the start. The real value comes when that traffic lands on your website and actually converts.
That’s where Pathmonk comes in.
When users click through from an LLM recommendation, they’re usually in the consideration or decision stage. They already trust your brand enough to visit. Now your job is to make their path to conversion frictionless, and personalized.
Pathmonk uses behavioral data and AI to instantly analyze a visitor’s intent, then delivers microexperiences tailored to what they’re looking for — without requiring coding or extra dev time.
For example:
- If a visitor shows high purchase intent, Pathmonk will streamline their experience to reduce friction — surfacing trust signals, simplifying the path to your primary CTA (like booking a tour or demo), and nudging them to act quickly.
- If the visitor is in early-stage research mode, Pathmonk won’t push for a hard conversion right away. Instead, it might present educational content, social proof, or key differentiators that build trust and move them one step closer to converting — without overwhelming them.
Pathmonk works in the background of your website, analyzing each visitor’s behavior in real time to understand their intent, no need for tracking cookies or manual setup.
Here’s how it works:
1. Behavioral signals are tracked instantly
As soon as a visitor lands on your site, Pathmonk starts analyzing how they interact: what pages they view, how long they stay, where they click, and how they navigate. These actions help detect whether someone is just browsing, comparing options, or ready to convert
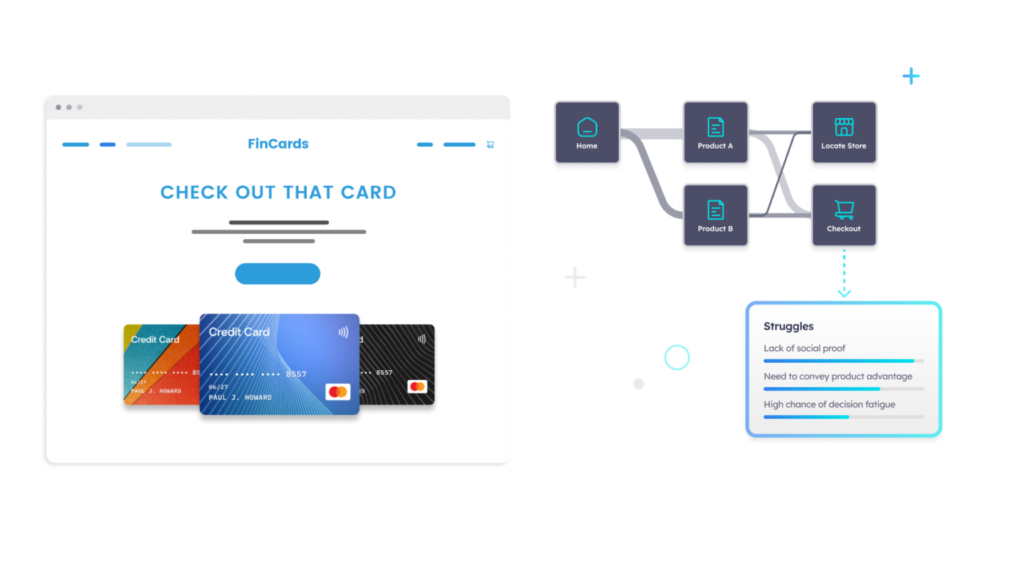
2. AI determines the visitor’s intent stage
Pathmonk’s AI model maps each user’s behavior to a specific stage in the buying journey, either awareness, consideration, or decision. This stage determines what kind of experience they’ll get.
3. Microexperiences are shown dynamically
Based on the intent, Pathmonk triggers a personalized microexperience, a small, non-intrusive interaction embedded into your site.
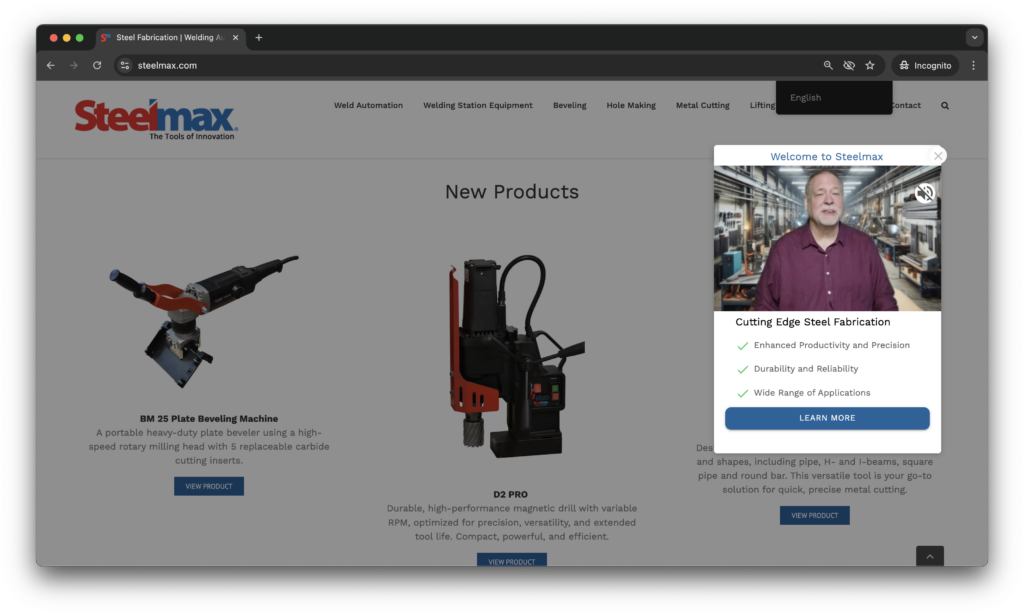
It could be:
- A subtle message highlighting your unique selling points
A tailored testimonial or case study - A personalized CTA pushing your main goal (e.g., book a demo, sign up, start trial)
The visitor sees one clear goal, but the supporting content adapts to what they’re likely to care about most.
4. No code, no slowdown
All of this runs in the background without slowing your site. You define your main goal, and Pathmonk handles the logic, delivery, and continuous optimization — no dev work required.
Increase +180%
leads
demos
sales
bookings
from your website with AI
Get more conversions from your existing website traffic delivering personalized experiences.
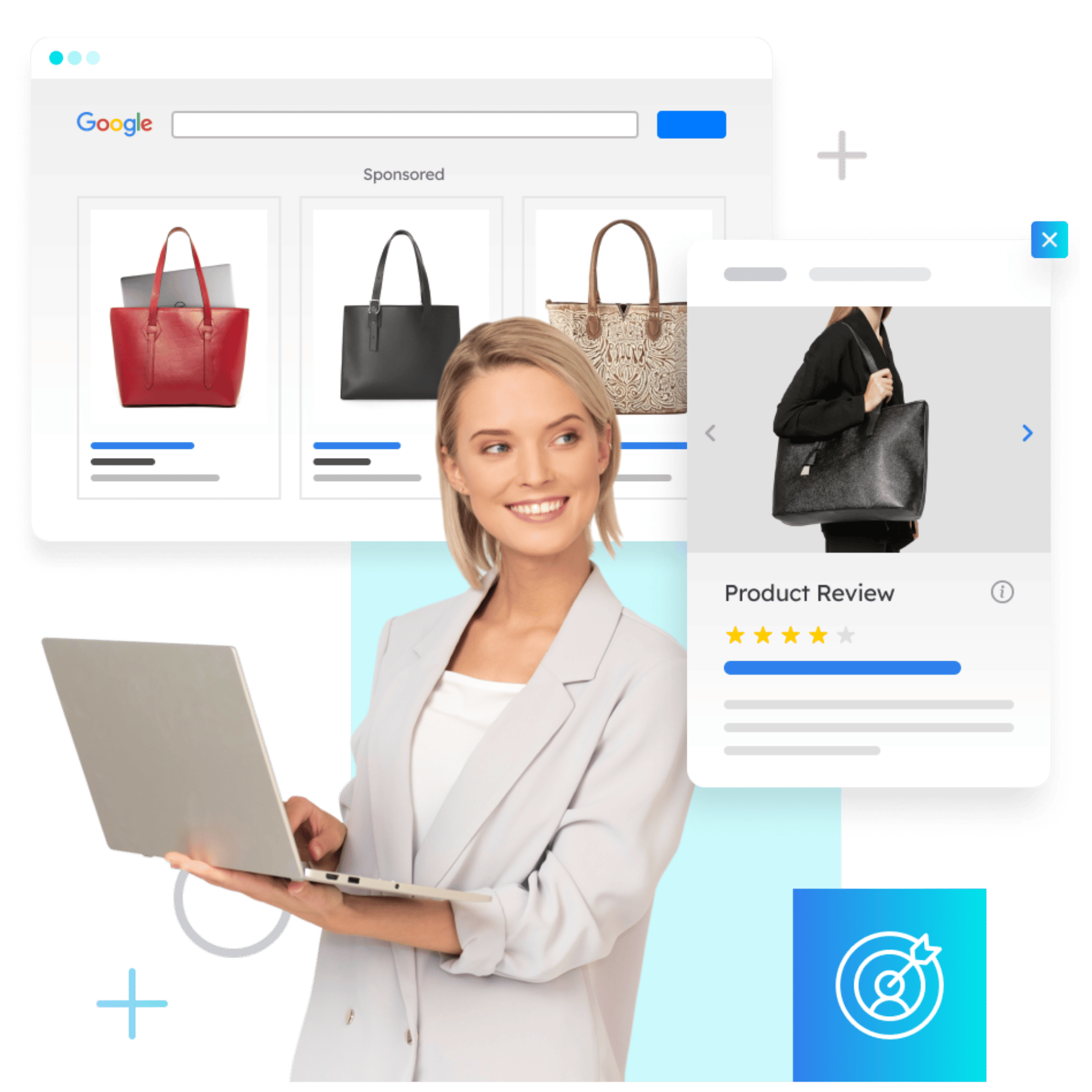