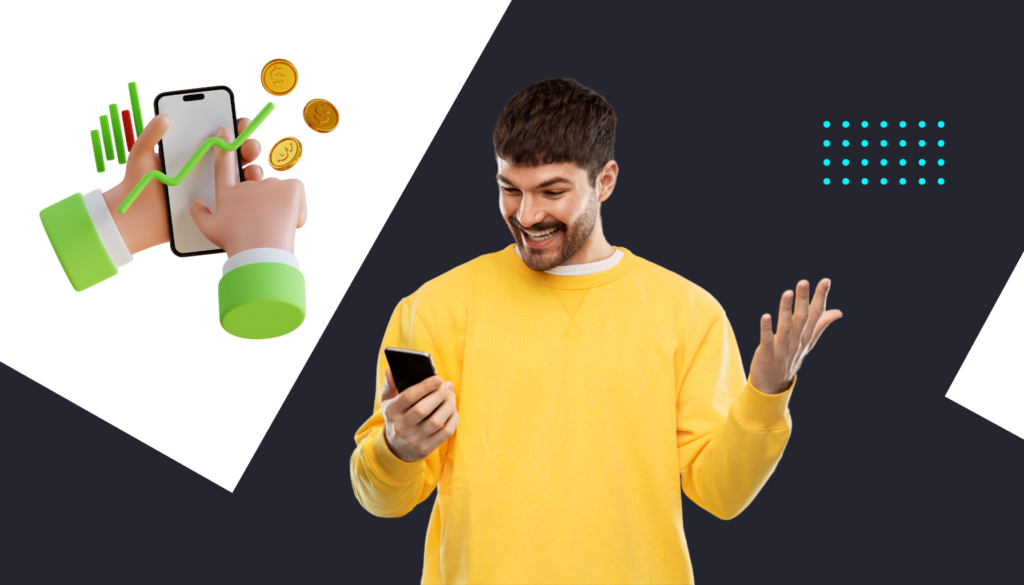
From retail to finance, healthcare, technology, and education, industries are recognizing the transformative potential of customer behavior data. It serves as a powerful tool that enables personalized experiences, operational efficiencies, and predictive capabilities, all aimed at meeting the nuanced demands of the modern consumer. This integration of data analytics transcends traditional boundaries, offering insights that lead to more informed decisions, enhanced customer engagement, and ultimately, stronger industry growth and development.
As businesses and institutions seek to navigate an increasingly competitive and complex landscape, the ability to harness, analyze, and act on data has become indispensable. This data-driven approach is not merely a technological advancement; it represents a fundamental shift in how organizations understand their customers, tailor their offerings and innovate for the future.
Table of Contents
The Power of Customer Behavior Data
In an era where data is more valuable than ever, the emphasis on customer behavior data stands out as a beacon for businesses aiming to navigate the competitive landscape with precision and insight. This kind of data encompasses the patterns, habits, preferences, and interactions customers exhibit across various touchpoints with a brand. Analyzing these insights allows businesses to understand their audience on a deeper level, tailor their offerings, and ultimately drive their decision-making processes toward success.
Core Principles and Significance
At its core, customer behavior data analysis involves systematically studying how customers interact with a business’s products, services, and overall brand. This could mean anything from tracking online browsing habits on a website and analyzing purchase history to monitoring social media engagement patterns. The significance of this analysis cannot be overstated, as it provides a treasure trove of actionable insights that can inform a wide array of business decisions.
The importance of analyzing customer data lies in its ability to transform abstract interactions into concrete, actionable insights. By understanding the ‘why’ behind customer actions, businesses can predict future behavior, identify opportunities for improvement, and personalize experiences at an individual level. This not only enhances customer satisfaction and loyalty but also optimizes business operations, leading to increased efficiency and profitability.
In essence, customer data is the foundation for developing a customer-centric strategy. It enables businesses to move beyond generic market segments and demographic profiles, offering a nuanced understanding of their audience’s desires, pain points, and preferences. This understanding is crucial for crafting compelling marketing messages, developing products that truly meet customer needs, and delivering services that exceed expectations.
Impact of Customer Behavior Data in the Retail Industry
In the dynamic world of retail, understanding and leveraging customer behavior data is more than a strategic asset; it’s a transformative force that reshapes every facet of the shopping experience. From personalized engagements to streamlined operations, data-driven insights into customer preferences, purchasing patterns, and engagement behaviors offer unparalleled opportunities for retailers to not only meet but exceed consumer expectations.
Personalizing the Shopping Experience
The personalization of the shopping experience, powered by in-depth analysis of customer behavior data, stands at the forefront of retail innovation. This strategy involves tailoring every interaction based on the individual customer’s history, preferences, and behavior. For instance, e-commerce platforms utilize browsing and purchase data to create a customized shopping environment for each user, displaying products, deals, and content that align with their interests. This level of personalization extends to physical stores, where retailers use loyalty programs and mobile app data to offer personalized discounts or suggestions through push notifications when the customer is near or in the store.
This hyper-personalized approach not only enhances customer satisfaction but also significantly boosts conversion rates and loyalty. The key lies in the retailer’s ability to anticipate needs and offer solutions before the customer even articulates them, creating a feeling of understood and valued personal connection.
Targeted Marketing and Product Placement
With customer behavior data, retailers can craft marketing strategies and product placements that hit the mark with precision. Targeted marketing campaigns use data analytics to identify specific customer segments and tailor messages that resonate on a personal level, dramatically increasing the effectiveness of advertising efforts. For example, segmentation can allow a retailer to identify and target “early adopters” with the latest product launches or offer exclusive previews to “high-value” customers, fostering brand loyalty and driving sales.
In the realm of product placement, both online and offline retailers can optimize layouts and displays to cater to the observed behaviors of their customers. Online, algorithms can adjust product listings and recommendations in real-time, guiding customers towards items they’re more likely to purchase. In physical stores, insights from data analytics can influence everything from shelf placement to in-store signage, strategically guiding customers through a path that maximizes product discovery and purchase likelihood.
Inventory and Supply Chain Optimization
Perhaps one of the most impactful uses of customer behavior data lies in the optimization of inventory management and supply chains. By predicting purchasing trends, seasonal demands, and potential supply chain disruptions through historical and real-time data analysis, retailers can maintain the right balance of stock levels, minimizing both overstocks and stockouts. This level of optimization not only reduces operational costs but also ensures that customer expectations for product availability are consistently met, thereby enhancing customer satisfaction and loyalty.
Advanced data analytics tools enable retailers to model various demand scenarios and adjust their supply chain strategies accordingly. For example, predictive analytics can forecast the popularity of specific items, allowing retailers to adjust their manufacturing orders and distribution plans to ensure that popular items are readily available across all channels.
Examples of Retail Innovation Through Customer Behavior Data
- Sephora’s use of data to create a highly personalized shopping experience both online and in-store, including tailored beauty recommendations and virtual try-on features, has set a benchmark in the beauty industry. Their ability to use data to understand each customer’s unique beauty profile and preferences demonstrates the power of personalized marketing and product development.
- Grocery chains, like Kroger, leverage customer data to optimize everything from product assortment to promotional offers, even customizing store layouts based on local customer preferences and purchasing habits. This localized approach to inventory and marketing significantly enhances the shopping experience, making it more relevant and convenient for customers.
- IKEA’s use of augmented reality apps to allow customers to visualize furniture in their homes before making a purchase is another innovative use of data to enhance customer decision-making and satisfaction. By understanding the importance of context and visualization in the furniture buying process, IKEA uses technology and data to solve a common customer pain point, thereby improving the overall shopping experience.
Transforming the Financial Services Industry through Customer Data
The financial services industry stands at a transformative juncture, powered by the unprecedented capabilities of customer data analytics. This shift towards a data-centric approach is not just enhancing operational efficiencies but is fundamentally altering how financial products are designed, how risk is managed, and how predictive analytics can safeguard and personalize the customer journey. The use of customer behavior data in the financial sector is leading to more tailored, responsive, and secure services that meet the evolving needs of consumers.
Custom Financial Products
The era of one-size-fits-all financial products is coming to an end, thanks to the insights gleaned from customer behavior data. Financial institutions are now able to design custom financial products that cater to the unique needs and circumstances of their customers. By analyzing transaction histories, savings patterns, and investment behaviors, banks, and financial service providers can offer personalized loan products, savings plans, and investment strategies that align closely with the individual’s financial goals and risk tolerance.
For example, a bank might use data analytics to identify customers with a high propensity to invest in sustainable projects. This insight allows the bank to offer green bonds or eco-friendly investment portfolios specifically to these customers, thereby not only meeting their investment criteria but also reinforcing the bank’s commitment to environmental sustainability.
Enhancing Risk Management
Customer behavior data is revolutionizing the way financial institutions manage risk. By leveraging detailed insights into customers’ financial behaviors, institutions can more accurately assess creditworthiness and predict potential defaults. This goes beyond traditional credit scoring models by incorporating a broader array of data points, including spending habits, income stability, and even social media activity, to paint a more comprehensive picture of risk.
Furthermore, this data-driven approach enables financial services to dynamically adjust their risk models in response to changing economic conditions, regulatory environments, and emerging risk factors. This agility in risk management not only protects the institution but also ensures that customers are offered credit and financial products that are appropriate to their capacity and needs, thereby reducing the chances of financial distress.
Predictive Analytics for Credit and Fraud
The application of predictive analytics in credit decision-making and fraud detection represents one of the most impactful uses of customer behavior data in the financial sector. By analyzing patterns in transaction data, financial institutions can identify early signs of fraud, such as unusual purchasing behavior or anomalous transactions, allowing for swift action to prevent losses.
Predictive analytics enables lenders to forecast future financial behavior based on past actions, improving the accuracy of creditworthiness assessments. This not only streamlines the credit approval process but also opens up opportunities for underbanked or unbanked individuals who may lack a traditional credit history but demonstrate financial reliability through other behaviors.
Examples of Financial Services Innovation Through Customer Behavior Data
- American Express uses advanced analytics to monitor cardholder transactions in real-time, allowing them to identify and react to fraudulent activity swiftly, thus safeguarding customer accounts.
- YNAB (You Need A Budget) analyzes users’ spending patterns to provide customized budgeting advice and financial planning strategies, helping users achieve their financial goals more effectively.
- Fintech startups are utilizing alternative data — such as rent payments, utility bills, and even social media activity — to offer credit products to those with thin credit files or no traditional credit history. This not only expands access to financial services but also demonstrates a more holistic approach to assessing creditworthiness.
Innovations in Healthcare Through Customer Data
The healthcare industry is undergoing a profound transformation, driven by the integration of customer (patient) data into every aspect of care delivery and operational management. This data-centric revolution is paving the way for unprecedented advancements in personalized patient care, predictive health analytics, treatment customization, and operational efficiency. By leveraging insights from patient behavior, medical histories, and real-time health data, healthcare providers can offer more tailored, effective, and proactive care solutions.
Personalized Patient Care
The cornerstone of modern healthcare innovation lies in personalized patient care, which is made possible through the nuanced understanding of patient data. This approach goes beyond traditional one-size-fits-all treatment models, offering care plans that are tailored to the individual’s genetic makeup, lifestyle, and health history.
For example, wearable technology and mobile health apps collect data on daily activities, heart rate, sleep patterns, and more, providing healthcare professionals with a comprehensive view of a patient’s health status in real-time. This level of insight enables the creation of highly personalized healthcare plans that can more effectively address the patient’s specific needs, potentially leading to better outcomes and enhanced quality of life.
Predictive Health Analytics and Treatment Customization
Predictive health analytics represent a game-changing application of customer data in healthcare, enabling the early detection of diseases and the customization of treatment plans. By analyzing patterns in patient data, including electronic health records, genomics, and lifestyle factors, healthcare providers can identify risk factors for diseases before symptoms appear. This predictive capability allows for the implementation of preventive measures, early interventions, and customized treatment plans that are optimized for the best possible outcomes.
For instance, machine learning models can sift through vast amounts of data to predict which patients are at risk of developing conditions like diabetes or heart disease, allowing healthcare providers to offer targeted advice on diet, exercise, and lifestyle changes to mitigate these risks.
Operational Efficiency and Outreach
The efficient operation of healthcare facilities is crucial for the delivery of timely and effective care. Customer data analytics play a vital role in enhancing operational efficiency and extending outreach efforts. By analyzing patient flow, appointment scheduling patterns, and resource utilization, hospitals and clinics can optimize their operations to reduce wait times, improve patient satisfaction, and allocate resources more effectively.
Moreover, data-driven insights can inform targeted outreach programs, identifying populations at risk and delivering personalized health education and preventive care recommendations. This proactive approach not only improves individual health outcomes but also has the potential to reduce the overall burden on the healthcare system.
Examples of Healthcare Innovation Through Customer Data
- Mayo Clinic’s use of data analytics to tailor treatment plans for cancer patients showcases the power of personalized medicine. By analyzing genetic data alongside traditional clinical data, they can identify the most effective treatment protocols for individual patients, significantly improving success rates.
- Telehealth platforms, which have seen exponential growth, rely heavily on patient data to provide remote care. These platforms use data from wearable devices and patient-reported symptoms to monitor health conditions in real-time, allowing for timely medical advice and interventions, thus making healthcare more accessible.
- Kaiser Permanente utilizes predictive analytics to screen large patient populations for chronic disease risk factors, enabling early intervention strategies that can prevent the onset of diseases or manage their progression more effectively.
Technology Sector’s Use of Customer Data
The technology sector stands at the forefront of utilizing customer data to foster innovation, enhance user engagement, and streamline user experiences. In an industry that thrives on rapid evolution and user-centric design, the insights derived from customer behavior data are invaluable. They not only inform product development but also enable companies to create feedback loops and optimize user experiences in ways that were previously unimaginable.
Driving Product Development and Innovation
Customer data acts as a beacon, guiding technology companies toward solutions that truly meet user needs. By analyzing usage patterns, feature adoption rates, and user preferences, tech companies can identify gaps in the market and develop new products or improve existing ones to fill those gaps. This data-driven approach ensures that innovation is not just about creating new technology but about solving real problems for users.
For example, streaming services like Netflix and Spotify use customer viewing and listening habits to inform the development of new features and algorithms for personalized recommendations. This not only enhances user satisfaction but also keeps these platforms at the cutting edge of technology and entertainment.
User Engagement and Feedback Loops
Customer behavior data is pivotal in creating effective user engagement strategies and establishing feedback loops that foster continuous improvement. Technology companies often employ analytics tools to monitor how users interact with their products, identifying which features are most popular and which may require reevaluation.
Feedback loops, facilitated through user surveys, in-app feedback tools, and social media monitoring, allow companies to gather direct input from their users. This feedback is then analyzed alongside behavioral data to prioritize updates and features that will deliver the most value. Such an approach ensures that product evolution is aligned with user expectations and needs, thereby fostering a loyal user base.
Enhancing User Experience with Pathmonk Intelligence
Pathmonk Intelligence represents a cutting-edge application of customer data analytics aimed at enhancing user experiences on digital platforms. By leveraging artificial intelligence and machine learning, Pathmonk Intelligence analyzes user behavior in real time to optimize website and app interfaces dynamically. This means that the layout, messaging, and calls-to-action can be adjusted automatically to suit the preferences and behaviors of each visitor, significantly boosting conversion rates and user satisfaction.
For instance, an e-commerce platform utilizing Pathmonk Intelligence could automatically tailor the browsing experience by highlighting products or offers that align with a visitor’s past behavior, search queries, and engagement metrics. This not only makes the shopping experience more personalized but also streamlines the path to purchase, benefiting both the user and the business.
Understand your customer journey analytics
See how your users behave, find drop-offs, and receive actionable insights with AI.
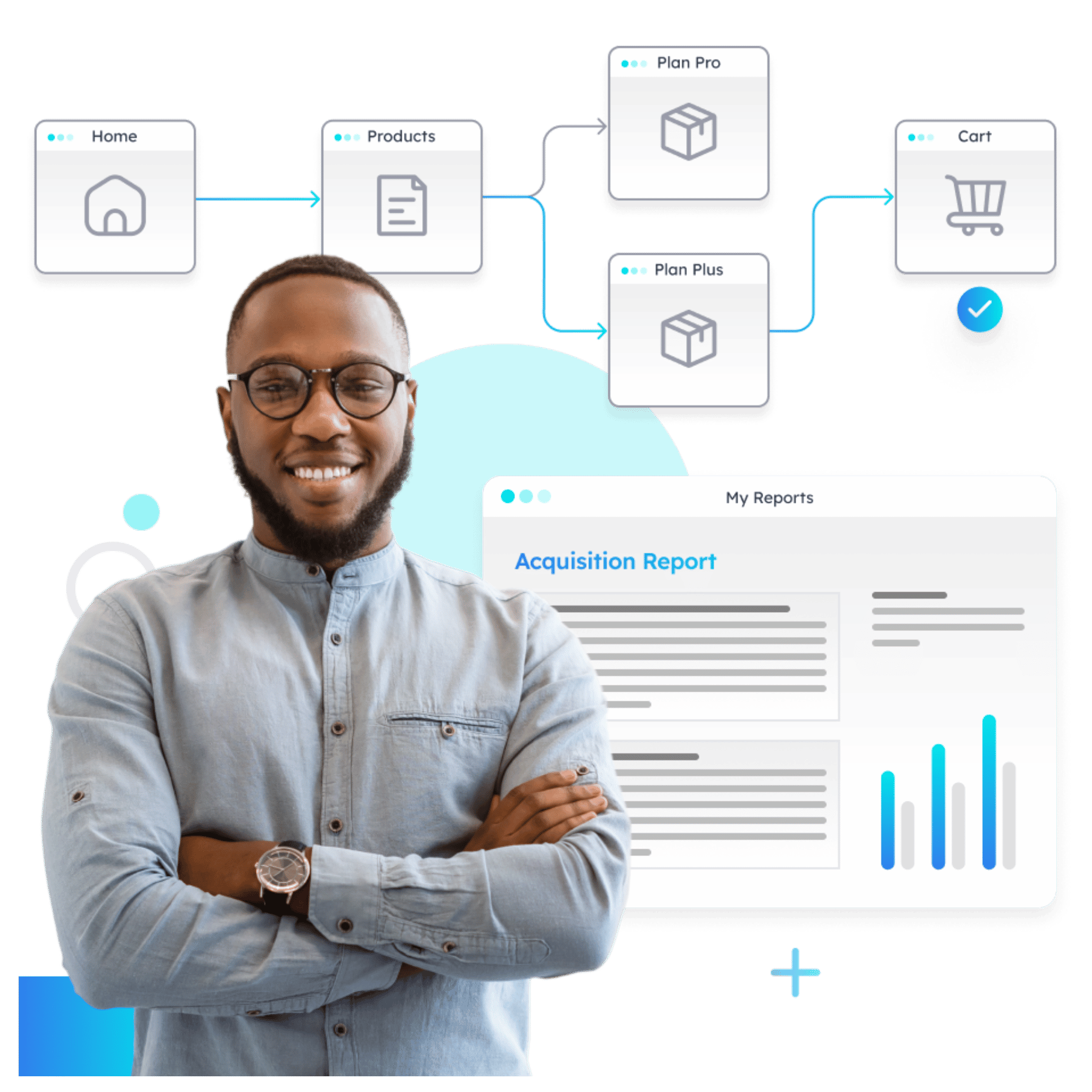
Examples of Technology Sector Innovation Through Customer Data
- Google and Apple use customer data to continuously refine their products and services. Google’s search algorithms are constantly updated based on user search behaviors and feedback, ensuring the most relevant and high-quality results. Apple uses customer usage data to improve the user interface and features of its devices and software, ensuring that each update is responsive to the needs of its users.
- Facebook and Instagram use sophisticated data analytics to curate personalized feeds, suggest connections, and target advertisements, enhancing user engagement and satisfaction.
Personalizing Education with Data Analysis
The education sector is experiencing a paradigm shift, moving towards personalized learning experiences that cater to the unique needs, abilities, and interests of each student. This shift is largely driven by the strategic use of data analysis, which enables educators and institutions to create adaptive learning environments, tailor educational content, and closely monitor student engagement and performance. The insights gained from this data are instrumental in designing education systems that are more effective, engaging, and inclusive.
Adaptive Learning Environments
Adaptive learning environments leverage data analytics to adjust the learning experience in real-time, based on the student’s progress, strengths, and areas for improvement. This approach ensures that each student receives a customized learning journey that is not only aligned with their current level of understanding but also challenges them appropriately to achieve their full potential.
Tailoring Educational Content
At the heart of adaptive learning environments is the capability to tailor educational content to meet the individual needs of each student. By analyzing data on students’ interactions with the material, quiz scores, and time spent on various topics, educational platforms can dynamically adjust the content, pace, and complexity of the curriculum. This means that students who grasp concepts quickly can be presented with more advanced material, while those who need more time can receive additional resources and support to solidify their understanding.
Student Engagement and Performance Analytics
Data analysis plays a crucial role in monitoring and enhancing student engagement and performance. By tracking metrics such as attendance, participation in discussions, submission of assignments, and performance on assessments, educators can identify patterns and trends that indicate how well students are engaging with the course material. This information is invaluable for identifying students who may be at risk of falling behind, enabling timely interventions to support their learning.
Performance analytics also offer insights into the effectiveness of teaching methods and materials, allowing educators to refine their approaches for better outcomes. This continuous feedback loop between student performance data and instructional strategies is key to creating a more responsive and effective educational experience.
Examples of Education Innovation Through Customer Behavior Data
- Khan Academy and Coursera use data analytics to provide personalized learning paths for students, offering recommended courses and exercises based on their progress and interests.
- Schools and universities are also adopting learning management systems that integrate data analytics to track student engagement across digital platforms, allowing educators to tailor their teaching strategies to the needs of their students. This can include modifying lesson plans, providing additional resources, or adopting different instructional techniques to improve engagement and comprehension.
- DreamBox Learning utilizes adaptive learning technologies to offer math education that adjusts to the learner’s responses in real time, providing a truly personalized learning experience that promotes mastery of mathematical concepts at the student’s own pace.
Conclusions
The integration of data analytics across retail, finance, healthcare, technology, and education showcases a universal shift towards personalized and efficient service delivery. This strategic embrace of customer behavior data is transforming industries by enhancing personalization, optimizing operations, and driving innovation.
In retail, personalized shopping experiences and inventory optimization are setting new standards for consumer engagement. Finance benefits from tailored products, improved risk management, and fraud detection, broadening access and security. Healthcare sees advancements in personalized care and predictive analytics, promising better outcomes. The technology sector leverages data for product development and user engagement, leading market innovation. Education uses analytics for customized learning paths and engagement improvement, aiming for more effective and inclusive experiences.
This cross-industry adoption of data analytics underlines the growing importance of deeply understanding and responding to customer needs. The future of these sectors will increasingly rely on leveraging data insights for innovation, efficiency, and enhanced customer satisfaction, marking a significant shift toward data-driven decision-making and service delivery.