Predictive Lead Scoring: Identifying High-Value Prospects with AI
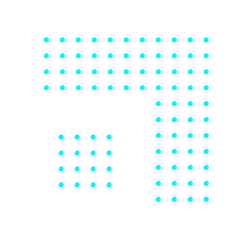
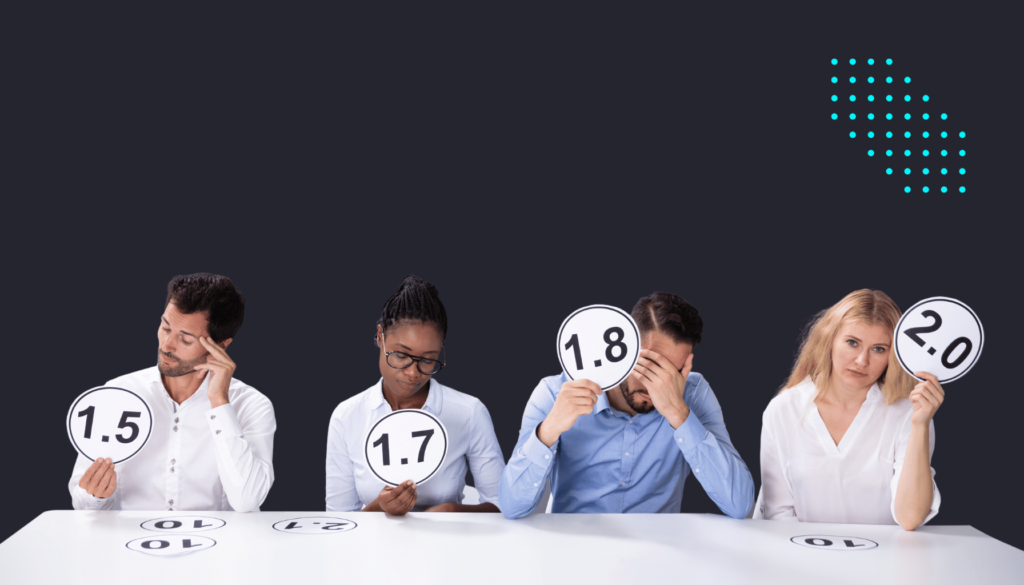
Effective lead generation is the lifeblood of successful marketing campaigns. However, the traditional methods of lead scoring, relying on manual assessments and limited data, often fall short in identifying the most promising prospects. This is where the power of artificial intelligence (AI) becomes a powerful tool. Predictive lead scoring, driven by advanced machine learning algorithms and data analytics, has revolutionized the way businesses identify high-value prospects.
We’ll dive deep into the world of predictive lead scoring and explore how AI is reshaping lead-generation strategies. We’ll uncover the inner workings of this cutting-edge technology, its benefits, data sources, machine learning models, implementation strategies, and more. By the end of this journey, you’ll have a comprehensive understanding of how AI can transform your lead-generation efforts and help you connect with the prospects most likely to convert into loyal customers.
What is Predictive Lead Scoring?
Predictive lead scoring is a sophisticated data-driven technique used by businesses to evaluate and rank potential leads based on their likelihood to convert into paying customers. Unlike traditional lead scoring methods that rely on manual rule-based criteria, predictive lead scoring leverages the power of artificial intelligence and machine learning to analyze vast datasets and uncover hidden patterns.
At its core, predictive lead scoring uses historical data on leads and customers to build predictive models. These models take into account a wide range of factors, including demographic information, online behavior, engagement history, and more. By examining these factors and their correlations with conversion outcomes, AI algorithms generate predictive scores for each lead. These scores provide valuable insights into which leads are most likely to make a purchase or take a desired action.
Here are the key differences with traditional lead scoring:
- Data-Driven vs. Rule-Based: Traditional lead scoring typically relies on predefined rules and static scoring criteria. In contrast, predictive lead scoring uses machine learning algorithms to analyze historical data and dynamically adapt to changing market conditions and customer behavior.
- Complexity and Accuracy: Predictive lead scoring models can handle a vast array of data sources and complex interactions, allowing for a more accurate assessment of lead quality. Traditional scoring may struggle to capture nuanced differences between leads.
- Continuous Learning: Predictive models continually learn and improve over time as they process new data. This adaptability ensures that lead scoring remains effective in the face of evolving customer preferences and market dynamics.
The Underlying Technology: Machine Learning and AI
The driving force behind predictive lead scoring is artificial intelligence and machine learning. These technologies enable you to harness the full potential of your data and extract actionable insights. Here’s how they work in this context:
- Machine Learning Algorithms: Predictive lead scoring employs a variety of machine learning algorithms, such as logistic regression, decision trees, random forests, or neural networks, to analyze data patterns. These algorithms identify which features (e.g. lead demographics, and website interactions) are most predictive of conversion.
- Feature Engineering: Data scientists and marketers work together to select and engineer relevant features (data attributes) that feed into the predictive models. Feature engineering is crucial for ensuring the models have the right inputs to make accurate predictions.
- Training and Validation: The predictive models are trained on historical data, using a portion of the data to learn patterns and another portion to validate their accuracy. This iterative process refines the models until they achieve a high level of prediction accuracy.
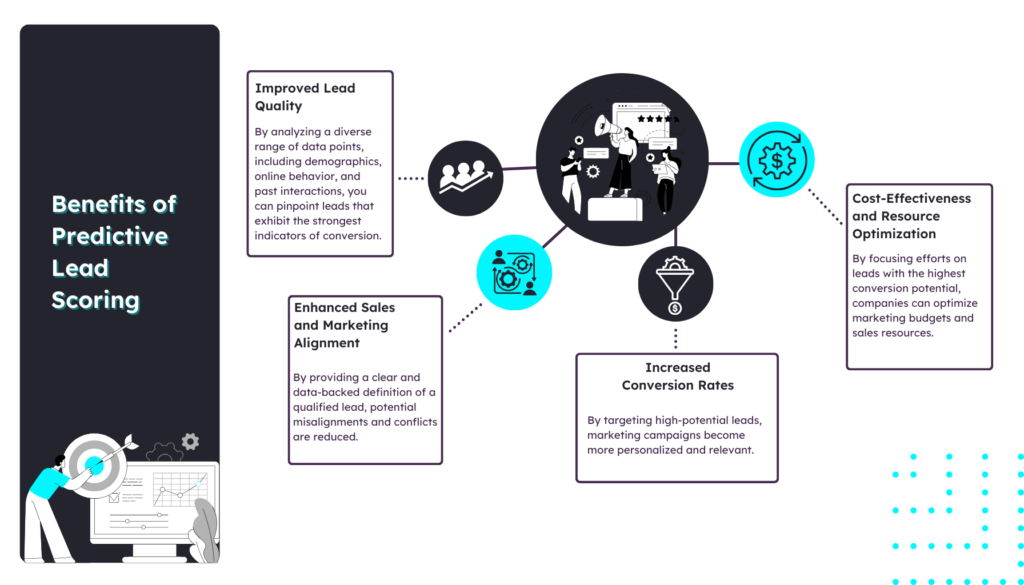
Benefits of Predictive Lead Scoring
The benefits of predictive lead scoring extend far beyond lead generation. It fundamentally transforms how you approach your marketing and sales efforts, leading to higher quality leads, improved collaboration between teams, higher conversion rates, and a more efficient use of resources. By harnessing the power of AI-driven lead scoring, you gain a competitive edge in a crowded marketplace.
1. Improved Lead Quality
Predictive lead scoring revolutionizes lead generation by prioritizing quality over quantity. By analyzing a diverse range of data points, including demographics, online behavior, and past interactions, you can pinpoint leads that exhibit the strongest indicators of conversion. This means marketing and sales teams are working with leads that are not only more likely to convert but also align with the ideal customer profile. As a result, resources are allocated more efficiently, leading to higher close rates and a more streamlined sales process.
2. Enhanced Sales and Marketing Alignment
Predictive lead scoring fosters greater collaboration between sales and marketing teams. By providing a clear and data-backed definition of a qualified lead, potential misalignments and conflicts are reduced. Both teams can align their strategies and efforts toward pursuing leads that are most likely to convert. This synergy leads to a more efficient and effective lead-handling process, ultimately driving revenue growth.
3. Increased Conversion Rates
One of the most compelling advantages of predictive lead scoring is its ability to significantly boost conversion rates. By targeting high-potential leads, marketing campaigns become more personalized and relevant. This tailored approach resonates with prospects, increasing the likelihood of them taking the desired action, whether it’s making a purchase, signing up for a trial, or requesting more information. The result is a substantial improvement in overall conversion metrics.
4. Cost-Effectiveness and Resource Optimization
Predictive lead scoring enables smarter resource allocation decisions. By focusing efforts on leads with the highest conversion potential, companies can optimize marketing budgets and sales resources. This means avoiding wasted resources on leads that are unlikely to convert, reducing the cost per acquisition, and ultimately improving the return on investment (ROI) of marketing campaigns. Additionally, predictive lead scoring can identify opportunities for nurturing lower-scoring leads, ensuring that no potential opportunity is overlooked.
Pathmonk Intelligence: Unveiling Cookieless Web Analytics for Lead Insights
Pathmonk Intelligence is a groundbreaking tool that redefines the way you gather insights about website visitors and leads, all while respecting privacy with cookieless web analytics. This innovative approach is particularly valuable in an era where privacy regulations are tightening and cookie-based tracking is becoming less effective.
This tool employs sophisticated predictive lead-scoring methods to analyze visitor behavior and patterns on your website. Here’s how it works:
- Visitor Identification: Pathmonk Intelligence analyzes the behavior of your website visitors, gathering data on their interactions, content consumption, and engagement.
- Predictive Lead Scoring: Leveraging AI and machine learning, Pathmonk Intelligence assigns predictive lead scores to each visitor. These scores indicate the likelihood of a visitor becoming a qualified lead or converting into a customer.
- Lead Insights: Pathmonk Intelligence provides detailed lead profiles that include information on a lead’s interests, preferences, and engagement history. This rich data allows you to tailor your marketing efforts and communication to the specific needs and interests of each lead.
- Segmentation: The tool enables you to segment your leads based on their predictive lead scores and behaviors. This segmentation is instrumental in crafting personalized marketing campaigns and nurturing sequences.
In essence, Pathmonk Intelligence empowers you to understand and engage with their website visitors more effectively. By combining predictive lead scoring with cookieless web analytics, you can deliver highly relevant and targeted content to your leads, increasing the chances of conversion.
Intuitive Cookieless Analytics for Your Web
Understand your customer journey, find drop-offs, and receive actionable insights with AI.
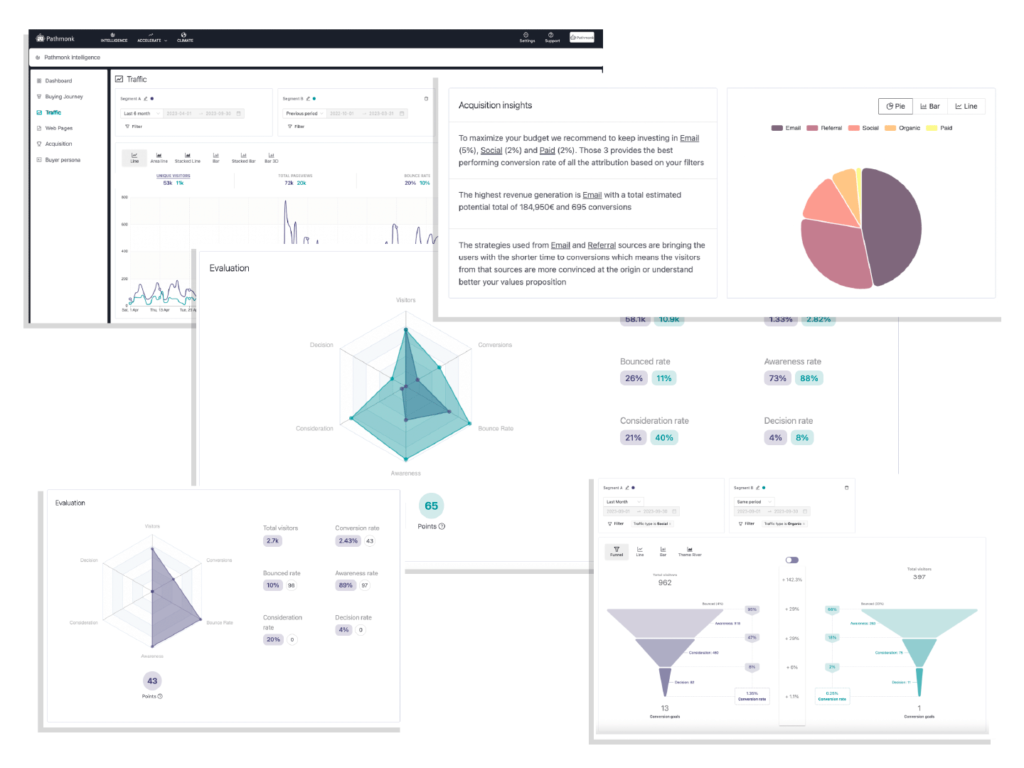
Pathmonk Accelerate: Elevating Web Experiences with AI-Powered Personalization
While Pathmonk Intelligence focuses on gathering insights, Pathmonk Accelerate takes the next step by using these insights to enhance website experiences and drive conversions. This tool uses AI-powered scoring generated by Pathmonk Intelligence to deliver automated and personalized website experiences to your website users.
Here’s how Pathmonk Accelerate achieves this:
- Real-time Scoring: Pathmonk Accelerate uses the predictive lead scores provided by Pathmonk Intelligence to assess a visitor’s intent and engagement in real time.
- Experience Personalization: Based on the visitor’s score and behavior, the tool dynamically adjusts website experiences, recommending relevant products, services, or content pieces. This ensures that each visitor receives messages tailored to their interests, increasing engagement.
- Call-to-Action Optimization: Pathmonk Accelerate optimizes the placement and visibility of call-to-action elements, such as contact forms or subscription prompts, to maximize conversion opportunities.
- Lead Nurturing: The tool can initiate automated lead nurturing sequences, delivering targeted interactions to guide visitors through the conversion funnel.
By combining predictive lead scoring from Pathmonk Intelligence with personalized web experiences offered by Pathmonk Accelerate, you can dramatically enhance your website conversion rates. Visitors receive content and interactions that resonate with their interests, leading to higher engagement and, ultimately, more conversions.
More Sales From Your Website With AI
Personalized interactions based on your users' behaviour to get +50% more conversions.
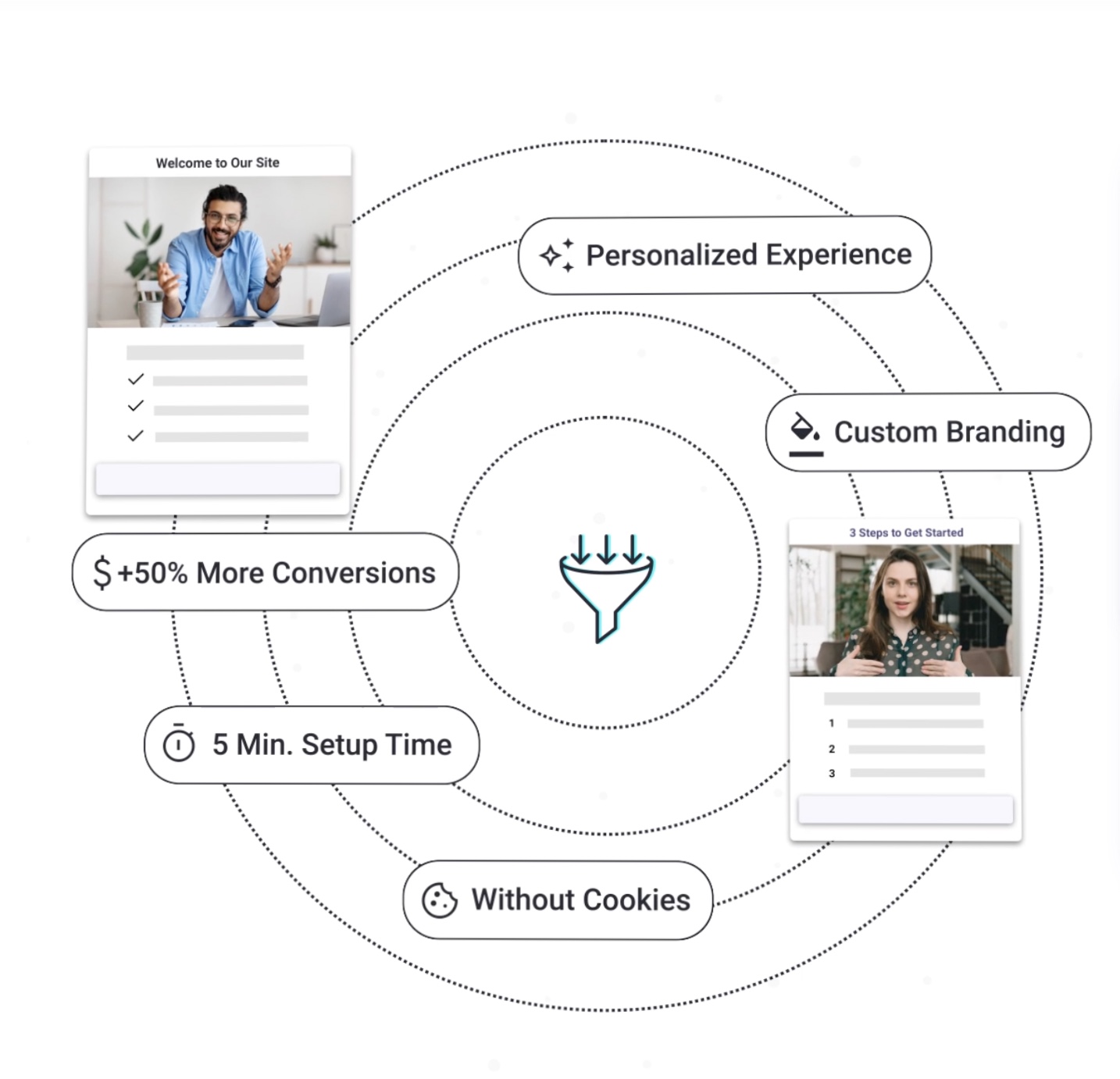
Data Sources for Predictive Lead Scoring
Data is the foundation of predictive lead scoring. Without a rich and diverse dataset, predictive models wouldn’t have the information they need to make accurate assessments of lead quality.
Here are the key types of data commonly used:
- Demographic Data: This includes information like age, gender, location, job title, and education. Demographics provide a foundation for understanding who your leads are.
- Firmographic Data: Especially important for B2B marketing, firmographic data covers details about a lead’s company, such as industry, company size, revenue, and location. It helps tailor your approach to the unique needs of customers.
- Behavioral Data: Behavioral data tracks how leads interact with your brand. This includes website visits, content downloads, email opens, clicks, and social media engagement. Behavioral data is particularly valuable for assessing a lead’s engagement and interest level.
- Technographic Data: In the B2B space, technographic data reveals the technologies and tools a company uses. This information can be used to identify compatibility or integration opportunities.
- Psychographic Data: This type of data delves into a lead’s personality traits, values, and interests. It helps in crafting messaging and offers that align with a lead’s psychographic profile.
- Historical Data: Historical data on lead and customer interactions provide insights into past behaviors, purchase history, and conversion paths. Analyzing this data helps in understanding trends and predicting future actions.
Machine Learning Models in Predictive Lead Scoring
Machine learning plays a pivotal role in predictive lead scoring, enabling you to build sophisticated models that can accurately predict lead conversion likelihood. As a marketer, you don’t have to worry about this specifically, but here’s an overview of some of the machine learning algorithms commonly used in this context for a better understanding of how they work:
- Logistic Regression: A widely used algorithm for binary classification problems, such as determining whether a lead will convert or not. It’s relatively interpretable and serves as a good starting point for lead-scoring models.
- Decision Trees: Decision trees are versatile and can handle both classification and regression tasks. They break down decisions into a series of if-else statements, making them interpretable and easy to visualize.
- Random Forests: Random forests are an ensemble of decision trees. They offer improved predictive performance by aggregating the predictions of multiple trees, reducing overfitting, and increasing accuracy.
- Gradient Boosting Models: Algorithms like XGBoost, LightGBM, and CatBoost are known for their exceptional predictive power. They build a strong predictive model by sequentially improving upon the weaknesses of the previous one.
- Neural Networks: Deep learning neural networks, particularly deep feedforward networks and recurrent neural networks (RNNs), can capture complex patterns in data. They excel at handling large datasets with numerous features.
- Support Vector Machines (SVMs): SVMs are effective for both classification and regression tasks. They aim to find the optimal hyperplane that maximizes the margin between classes, making them robust against overfitting.
- K-Nearest Neighbors (K-NN): K-NN is a simple yet effective algorithm that classifies leads based on the majority class of their k-nearest neighbors. It’s particularly useful when the underlying data distribution is non-linear.
Often, a combination of these algorithms or an ensemble approach is used to achieve the best results.
Best Practices for Predictive Lead Scoring
Predictive lead scoring is a powerful tool, but to fully harness its potential, it’s essential to follow best practices. Here are key recommendations for maximizing the effectiveness of your predictive lead-scoring efforts:
- Collaboration Between Teams: Encourage close collaboration between your marketing and sales teams. Ensure that they share insights and feedback to refine the lead scoring model continually.
- Clear Lead Definitions: Establish clear and well-defined criteria for what constitutes a qualified lead. Ensure that both marketing and sales teams have a shared understanding of lead quality.
- Regular Model Updates: Predictive lead scoring models should evolve with your business and market dynamics. Regularly update and retrain models to adapt to changing customer behavior.
- Feedback Loop: Implement a feedback loop to capture insights from the sales team about the quality of leads generated. This information can be used to refine the lead scoring criteria.
- Segmentation: Use the lead scores to create segmented marketing campaigns. Tailor your messaging and content to the unique needs and interests of each segment.
Conclusion
Predictive lead scoring, driven by AI and machine learning, empowers you to navigate the complex landscape of lead generation with unprecedented clarity. It doesn’t just identify high-value prospects; it understands them. It deciphers their preferences, anticipates their needs, and speaks to them on an individual level. In essence, it transforms faceless leads into valued relationships.
But this is not merely a technological advancement; it’s a paradigm shift. It’s a move away from traditional marketing methods that cast wide nets in the hope of capturing a few fish. Instead, it’s about cultivating genuine connections with prospects who are not just willing but eager to engage with your brand.
However, with this immense power comes great responsibility. As we leverage AI for predictive lead scoring, we must do so ethically and transparently. We must guard against biases, ensure data privacy, and maintain fairness in our scoring models. It’s a reminder that while AI can amplify our capabilities, it’s ultimately humans who determine how it’s wielded.
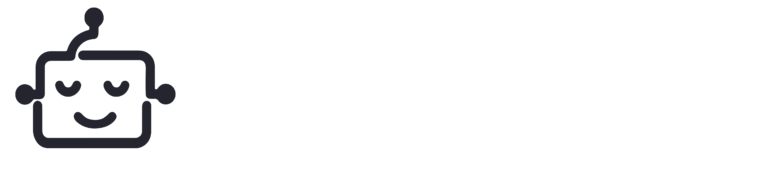
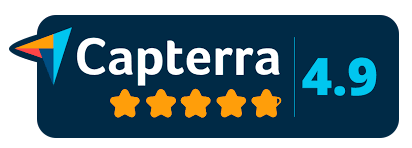
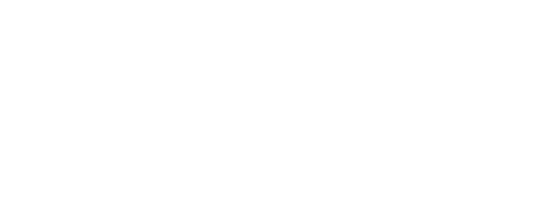
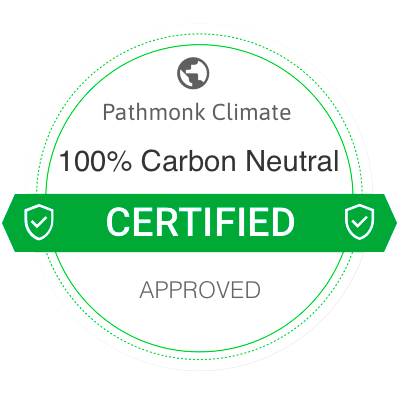