Overcoming Data Fragmentation in Cookieless Advertising
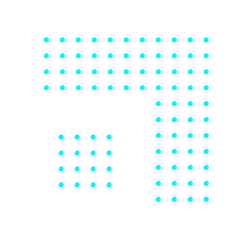
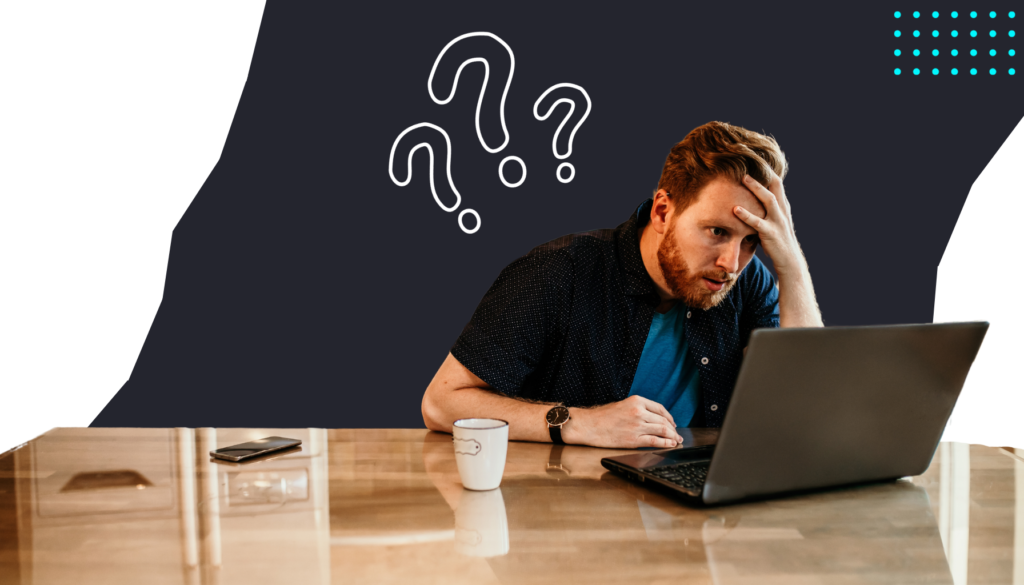
The digital advertising landscape is undergoing a significant transformation as the use of third-party cookies, which have been the backbone of data-driven advertising for years, is being phased out. This shift presents a major challenge for advertisers, as it hinders their ability to collect and utilize user data to deliver personalized and relevant ad experiences.
Data fragmentation, which occurs when data is spread across multiple silos and lacks consistency, is a prevalent issue in the current advertising ecosystem. This fragmentation can severely limit advertisers’ understanding of user behavior and make it difficult to effectively target ads. As a result, advertisers are facing a decline in ad performance and a reduced ability to connect with their target audience.
In this article, we’ll explore how to overcome these challenges and adopt new strategies and technologies to address data fragmentation.
Table of Contents
Understanding Data Fragmentation and the Phase Out of Cookies
Data fragmentation, a prevalent issue in the digital advertising landscape, refers to the dispersion of data across multiple silos, each with its own data format, structure, and ownership, making it increasingly challenging for advertisers to gather comprehensive insights into consumer behavior.
These are some common reasons why your data might be fragmented:
- Siloed Data: Data is compartmentalized and isolated within different departments, systems, or organizations, making it difficult to access and analyze a comprehensive view of customer behavior.
- Inconsistent Data Formats: Data is stored in different formats, making it challenging to integrate and analyze for meaningful insights. This can lead to data discrepancies and hinder the creation of accurate user profiles.
- Lack of Cross-Device Data Alignment: Data collected from different devices, such as smartphones, tablets, and desktops, is not linked consistently, making it difficult to track user behavior across their digital journey.
The impact of cookie deprecation on traditional advertising strategies
Traditionally, cookies served as the linchpin for tracking and collecting user data, providing advertisers with a somehow cohesive view of user interactions across the internet. However, as we witness the phasing out of cookies, data fragmentation has become a pressing issue. Advertisers are now faced with the challenge of adapting to a landscape where the familiar tools for user identification and tracking are no longer as pervasive.
Cookie deprecation disrupts the traditional funnel-based approach to advertising, impacting essential components such as retargeting, frequency capping, and cross-device tracking. Advertisers must now reconsider their methodologies to ensure they can still effectively reach and engage their target audiences without the crutch of cookies.
Moreover, the shift away from cookies requires a reevaluation of the effectiveness of third-party data sources. Advertisers need to explore alternative methods for obtaining valuable insights into consumer behavior and preferences, recognizing the limitations and challenges posed by the dwindling availability of third-party data.
The Challenges of Cookieless Advertising
1. Limited User Tracking and Targeting Capabilities
In the absence of cookies, advertisers face a substantial challenge in tracking user behavior across the digital landscape. Cookies traditionally allowed for the seamless tracking of users, enabling advertisers to understand their preferences, interests, and online activities. With the erosion of this tracking mechanism, there is a notable limitation in the ability to create a cohesive and granular profile of users.
The result is a reduction in the precision and accuracy of audience targeting. Advertisers must now contend with a fragmented view of user interactions, making it more difficult to tailor campaigns to specific demographics and interests. The challenge lies in finding alternative methods to effectively identify and engage with the right audience without relying on the comprehensive tracking that cookies once provided.
2. Disruption of Retargeting and Personalization Efforts
Retargeting, a fundamental strategy for re-engaging users who have previously interacted with a brand, is significantly affected by the cookieless environment. Cookies played a crucial role in tracking users across websites, allowing advertisers to deliver targeted ads based on their previous online behavior. With this capability diminished, retargeting efforts lose a degree of precision, potentially leading to less effective and more generic ad campaigns.
Moreover, the personalization of content, which was streamlined through cookie-driven insights into user preferences, now faces hurdles. Advertisers must explore alternative methods, such as first-party data and contextual signals, to deliver personalized content and maintain a high level of relevance in their advertising efforts.
3. Reduced Effectiveness of Attribution Modeling
Attribution modeling, a process that assigns value to different touchpoints in the customer journey, relies heavily on accurate data tracking. Cookies were pivotal in providing a seamless flow of user data, allowing advertisers to attribute conversions and interactions to specific channels and campaigns effectively. In a cookieless landscape, the reliability and granularity of attribution modeling face challenges.
The inability to track users consistently across their online journey makes it harder to determine the impact of each advertising touchpoint. Advertisers must adapt by reevaluating their attribution models, incorporating alternative data sources, and adopting more sophisticated methodologies, such as machine learning algorithms, to glean insights into the true impact of their advertising efforts.
Strategies for Overcoming Data Fragmentation
1. Embracing First-Party Data and Ethical Data Collection
In the era of cookieless advertising, establishing direct relationships with users becomes paramount. Advertisers should prioritize cultivating connections with their audience through transparent communication and value-driven interactions. This involves encouraging users to willingly share information, preferences, and interests, creating a foundation for robust first-party data.
Building direct relationships fosters trust, as users are more likely to share accurate and relevant information when they understand the value they receive in return. By engaging in personalized communication, feedback loops, and loyalty programs, advertisers can nurture a sense of community, making users more willing to provide the first-party data necessary for targeted advertising.
Ethical considerations are central to successful first-party data collection. Advertisers must prioritize user privacy, clearly communicate data usage policies, and provide users with the option to opt in or out of data collection practices. An ethical approach builds trust, mitigates concerns over privacy violations, and ensures the longevity of user engagement.
Strategies for ethical data collection include transparent consent forms, clear data usage statements, and giving users control over their preferences. Advertisers should adopt privacy-by-design principles, integrating ethical considerations into the very foundation of their data collection processes.
2. Leveraging Contextual Targeting
Contextual targeting emerges as a potent strategy in the absence of granular user tracking. Instead of relying on user-specific data, contextual targeting focuses on the content context in which ads are displayed. Advertisers can align their campaigns with the themes, topics, and sentiments of the content, ensuring relevance without compromising user privacy.
This approach involves analyzing the context of websites, articles, or videos to understand the audience’s interests. Advertisers can then tailor their messages to align seamlessly with the surrounding content, delivering a more organic and engaging advertising experience.
Benefits of Contextual Targeting
- Enhanced relevance: Ads align closely with the user’s current interests.
- Privacy-friendly: Doesn’t rely on individual user tracking.
- Flexibility: Adaptable to various content environments and platforms.
Challenges of Contextual Targeting
- Limited personalization: Lacks the depth of personalized targeting based on individual user behavior.
- Dynamic content: Requires continuous monitoring and adjustment to stay aligned with evolving content trends.
- Adapting to varied contexts: Differentiating between positive and negative content associations can be challenging.
3. Investing in AI and Machine Learning
Artificial Intelligence (AI) plays a pivotal role in mitigating data fragmentation by offering predictive modeling capabilities. Through machine learning algorithms, advertisers can analyze vast datasets to identify patterns, preferences, and trends. Predictive modeling enables the creation of robust audience segments, even in the absence of traditional tracking mechanisms.
AI-driven predictive modeling goes beyond simple demographics, incorporating behavioral and contextual signals to anticipate user interests. This enables advertisers to deliver more targeted and personalized content to their audience, enhancing the overall effectiveness of their campaigns.
How Machine Learning Algorithms Can Enhance Advertising Efficiency
- Dynamic targeting: Machine learning algorithms adapt in real-time, optimizing ad targeting based on user behavior and contextual changes.
- Improved decision-making: AI processes vast amounts of data quickly, enabling advertisers to make informed decisions on ad placements, creative elements, and bidding strategies.
- Attribution modeling: Machine learning enhances the accuracy of attribution models, providing a more nuanced understanding of the customer journey and the impact of each touchpoint.
As advertisers navigate the cookieless landscape, investing in AI and machine learning technologies becomes not only a necessity but a strategic advantage in crafting effective, data-driven advertising campaigns. These technologies empower advertisers to glean actionable insights, refine targeting strategies, and maintain a competitive edge in the evolving digital advertising ecosystem.
Pathmonk Intelligence: Unveiling Insights with Cookieless Analytics
In the wake of the cookieless revolution, Pathmonk Intelligence emerges as a powerful tool to overcome the data fragmentation that has plagued the advertising industry for years. Powered by advanced machine learning algorithms, Pathmonk Intelligence seamlessly works with first-party data from various sources, eliminating the reliance on third-party cookies and providing advertisers with a holistic view of user behavior. Want to give it a go? Try Pathmonk Intelligence for free and witness firsthand the transformative impact on your marketing endeavors.
Intuitive Cookieless Analytics for Your Web
Understand your customer journey, find drop-offs, and receive actionable insights with AI.
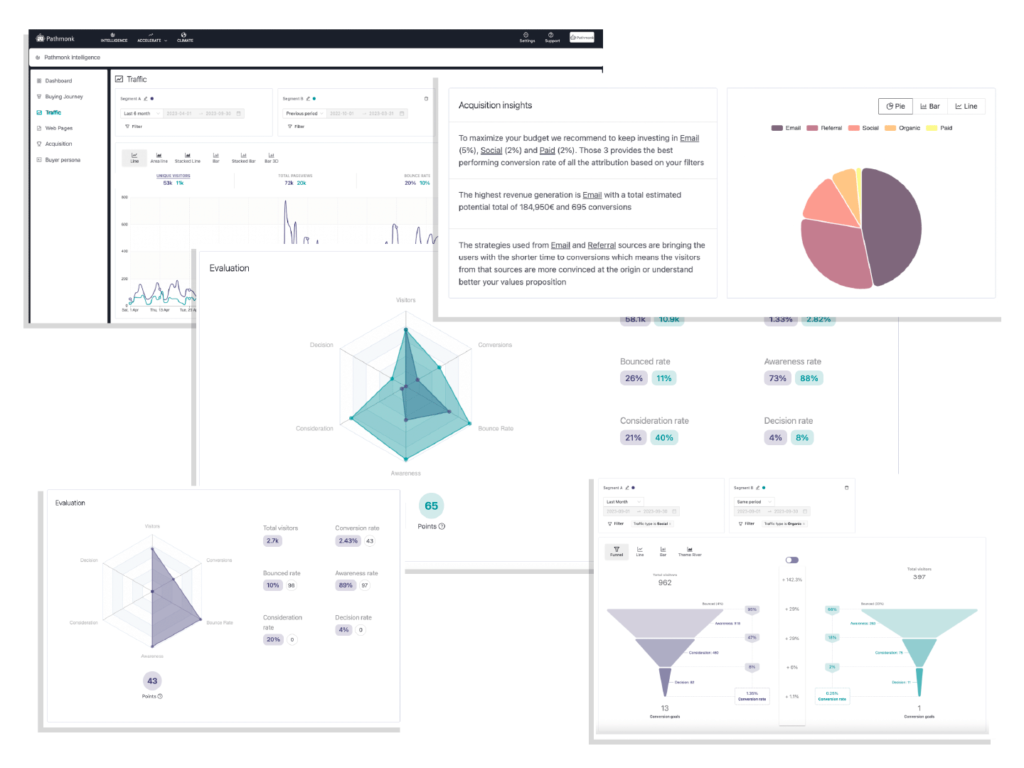
Data Reconciliation and Quality Assurance
Pathmonk Intelligence’s capabilities go beyond simple data consolidation. It proactively identifies and resolves data discrepancies, ensuring data quality and consistency. This meticulous approach ensures that advertisers are working with accurate and reliable information, enabling them to make informed decisions and optimize their campaigns effectively.
Unveiling Hidden Patterns and Insights
Pathmonk Intelligence delves into the depths of fragmented data, uncovering hidden patterns and insights that would otherwise remain elusive. Its ML algorithms analyze vast datasets, extracting meaningful correlations and trends that provide advertisers with a deeper understanding of user behavior and preferences. This granular level of insight empowers advertisers to tailor their messaging and targeting strategies with precision, maximizing ad engagement and driving conversions.
Predictive Analytics for Personalized Experiences
Pathmonk Intelligence’s predictive analytics capabilities take personalization to the next level. By analyzing historical data and current trends, it can accurately predict user behavior, enabling advertisers to deliver pre-emptive and relevant ad experiences. This proactive approach ensures that ads are always aligned with the user’s interests, fostering engagement and maximizing campaign performance.
Who Said Cookies?
Yes, Pathmonk Intelligences does all this completely independent from cookies. Unlike traditional methods that heavily rely on cookie-based tracking, Pathmonk Intelligence showcases that advertisers can achieve comprehensive insights into user behavior without the need for cookies.
Conclusion: A Cookieless Future Without Pain
As the cookieless era unfolds, data fragmentation poses a significant challenge for advertisers. However, with Pathmonk Intelligence, this obstacle can be overcome, enabling advertisers to deliver personalized and effective ad campaigns that resonate with their target audience.
Pathmonk Intelligence’s ML-powered solutions address data fragmentation, ensuring data quality, uncovering hidden insights, and providing predictive analytics – all without the need for cookies. This innovative approach empowers advertisers to thrive in the cookieless world and achieve sustainable growth in the digital advertising landscape.
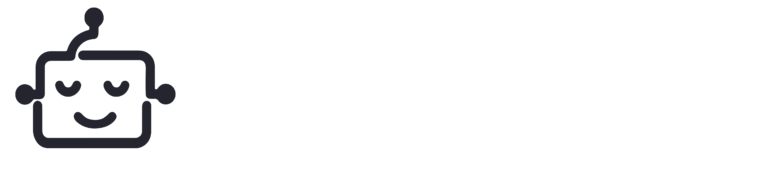
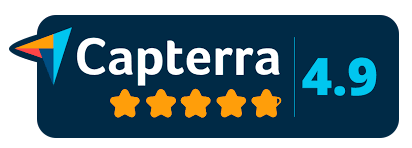
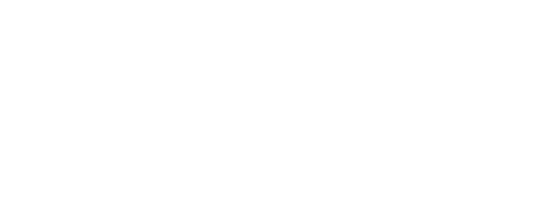
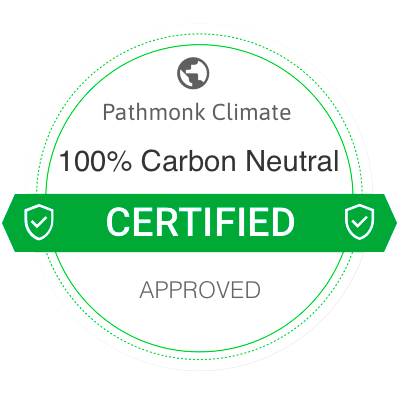